Top 5 Challenges AI Leaders Face Today
Explore the top challenges AI leaders face in 2025, from talent shortages to regulatory compliance, and discover actionable solutions.
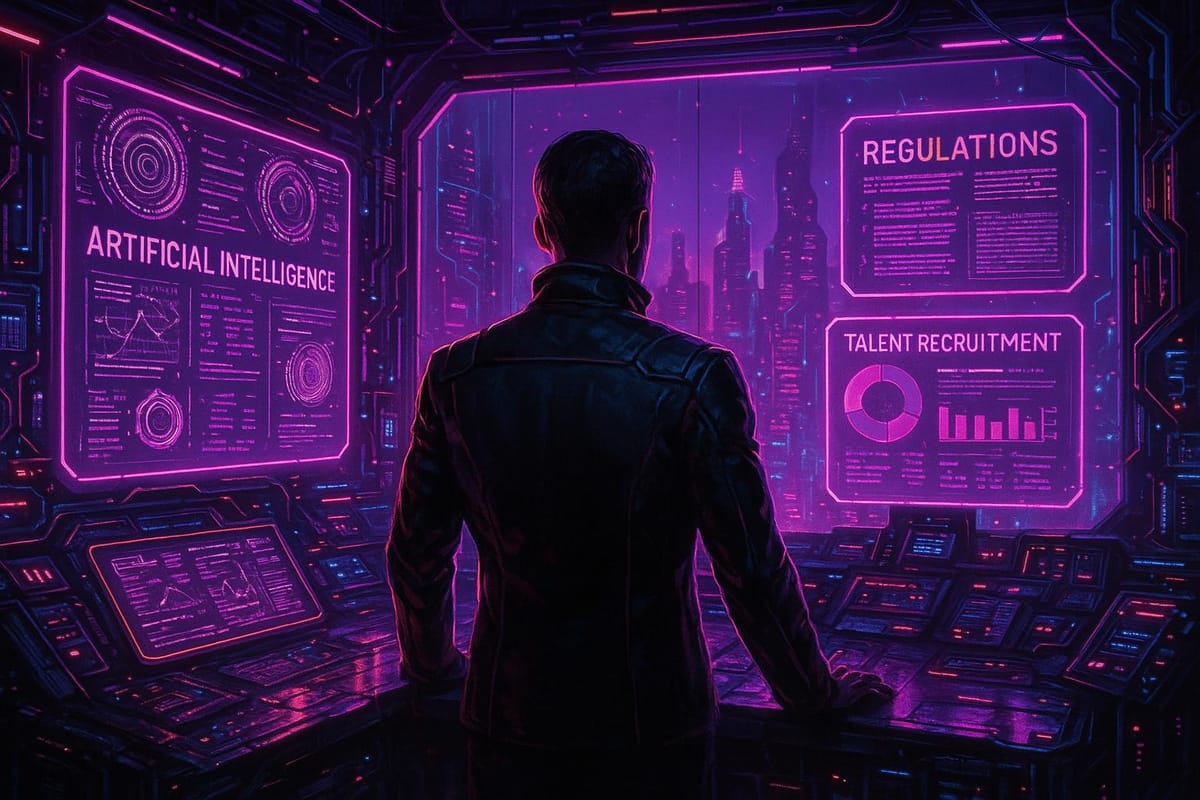
AI leaders face five key challenges in 2025:
- Talent Shortages: Finding and training AI experts is tough due to high demand and rapid tech changes.
- Building Responsible AI: Ensuring ethical, transparent, and unbiased AI systems is critical.
- Data Quality Issues: Poor data quality and tech infrastructure limitations hinder AI performance.
- Team Management: Leading teams through AI adoption requires training, collaboration, and clear communication.
- Regulatory Compliance: Navigating evolving AI regulations like GDPR and CCPA is complex and costly.
Quick Solutions:
- Upskill Teams: Invest in internal training and partnerships with AI consulting firms.
- Ethics First: Develop frameworks for fairness, transparency, and accountability.
- Improve Data Practices: Adopt strong data governance and scalable infrastructure.
- Support Teams: Provide training, clear documentation, and change management strategies.
- Stay Compliant: Build “compliance by design” into AI systems and conduct regular audits.
These strategies help organizations overcome hurdles and unlock AI’s full potential while staying competitive.
AI Leadership Challenges: 5 Key Questions
1. Finding and Training AI Talent
The demand for skilled AI professionals is outpacing supply. As organizations rush to implement AI solutions, McKinsey & Company's 2023 survey reveals that 26% of companies are using AI for contact center automation, while 23% are leveraging it for personalization efforts.
The challenges in this area fall into two main categories:
Recruitment Bottlenecks:
It’s tough to find specialists who can both create and integrate AI solutions - a combination of skills that’s not easy to come by.
Skills Development Hurdles:
Even when companies manage to hire AI experts, the rapid pace of technological change often makes their expertise outdated in no time.
These obstacles are pushing business leaders to rethink how they manage and nurture AI talent.
To tackle these issues, many organizations are turning to fresh talent strategies:
"The insights gained from NAITIVE AI advisory sessions have been enlightening." – Sofie, CEO
Internal Training Programs
Investing in internal training is becoming a priority for AI-driven companies. By providing documentation, structured learning, and ongoing support, they’re equipping their teams with the tools to stay ahead.
Strategic Partnerships
Collaborating with AI consulting firms has become a go-to solution for many. These partnerships offer several advantages:
- Access to specialized expertise without the cost of full-time hires
- Custom strategies tailored for specific AI goals
- Smooth integration of AI systems, while simultaneously upskilling internal teams
Knowledge Transfer
Forward-thinking AI leaders are emphasizing knowledge sharing. They ensure external consultants not only implement AI solutions but also train in-house teams to manage and improve these systems over time. This approach helps build long-term self-sufficiency while keeping operations aligned with evolving technologies.
2. Building Responsible AI Systems
Developing responsible AI systems is a pressing concern for today's AI leaders. As they tackle talent shortages and integration hurdles, ensuring ethical and transparent AI operations remains a cornerstone of their efforts. Creating such systems involves balancing technical execution with a commitment to fairness and accountability.
Ethical Framework Development
An ethical framework serves as the foundation for responsible AI development. It sets clear expectations and principles for how AI systems should operate.
Transparency Requirements:
- AI decisions should be explainable and easy to understand.
- System behaviors must be documented thoroughly.
- Regular audits and performance reviews are essential to maintain accountability.
Fairness Measures:
- Detect and address biases in AI algorithms.
- Ensure equitable treatment across all user groups.
- Conduct routine testing to identify and fix any discriminatory patterns.
While these frameworks provide a roadmap, implementing them effectively comes with its own set of obstacles.
Implementation Challenges
Data Quality Control:
The cornerstone of ethical AI is high-quality, representative data. To achieve this, AI leaders need to ensure their training data is:
- Free from historical and systemic biases.
- Properly sourced with clear documentation.
- Regularly updated and validated for accuracy.
System Integration:
Responsible AI systems must seamlessly integrate into existing infrastructures without compromising ethical standards. This involves:
- Implementing secure protocols for handling sensitive data.
- Using privacy-preserving technologies to protect user information.
- Continuously monitoring for performance and compliance with established guidelines.
These challenges highlight the importance of actionable strategies to build and maintain ethical AI systems.
Practical Solutions
Organizations like NAITIVE AI Consulting Agency have developed structured approaches to address these challenges. Their focus on responsible AI revolves around three crucial areas:
Focus Area | Implementation Strategy | Expected Outcome |
---|---|---|
Validation | Ongoing testing and debugging | Consistent and dependable results |
Monitoring | Real-time oversight of systems | Early identification of problems |
Adaptation | Quick responses to new issues | Long-term stability and growth |
Documentation and Training
Comprehensive documentation and consistent training are vital for upholding the principles of responsible AI. To achieve this, organizations should:
Create Clear Guidelines:
- Record all decision-making processes within AI systems.
- Develop and maintain protocols for system updates and changes.
Provide Continuous Support:
- Schedule regular training sessions to keep teams informed.
- Update documentation as systems evolve to reflect any changes.
- Establish clear reporting channels for employees to flag concerns or issues.
3. Managing Data Quality and Tech Limits
AI leaders often grapple with two major hurdles: ensuring high data quality and addressing the limitations of technical infrastructure. These challenges can significantly influence how effective and reliable AI systems are across an organization.
Data Quality Management
Low-quality data can lead to inaccurate predictions, biased outcomes, reduced system performance, and increased operational costs. To tackle these issues, organizations need to adopt strong data governance practices. This includes implementing automated data validation processes and maintaining rigorous data hygiene protocols to ensure the integrity and reliability of their datasets.
At the same time, having a solid technical foundation is equally important to make the most of these data assets.
Technical Infrastructure Challenges
For AI initiatives to succeed, organizations need a technical backbone that is both robust and scalable. However, many struggle with issues like:
- Processing Capabilities: Limited computing power, storage space, and network bandwidth can bottleneck AI performance.
- System Integration: Compatibility problems with legacy systems, challenges in managing APIs, and ensuring alignment with security protocols often complicate deployment.
Streamlining services to address these limitations can help organizations improve both performance and scalability.
Managed Service Solutions
To navigate these technical roadblocks, many organizations turn to managed service strategies. These services provide ongoing optimization and management, freeing up internal resources. For instance, Sarah Johnson, CXO, shared:
"The AI Agent NAITIVE designed now manages 77% of our L1-L2 client support".
Infrastructure Optimization
Building an infrastructure that balances complexity with functionality is key to effective AI implementation. Organizations can achieve this by focusing on specific strategies:
Component | Strategy | Outcome |
---|---|---|
Computing | Scalable cloud solutions | Flexible capacity |
Storage | Distributed systems | Better accessibility |
Network | Load-balanced architecture | Improved overall performance |
Performance Monitoring
Monitoring system performance is a must for maintaining efficiency. This involves tracking key metrics such as resource usage and system response times. Early detection of issues, combined with automated scaling, can help prevent disruptions. Regular updates to the system also ensure it stays efficient and aligned with evolving requirements.
Technical Scalability
As AI demands grow, organizations need infrastructures that can scale alongside them. John, CEO, remarked:
"The Voice AI Agent Solution NAITIVE implemented is from the future".
4. Leading Teams Through AI Adoption
Guiding organizations through the integration of AI involves navigating both technical challenges and the human side of change. Effective leadership in this space requires uniting teams around the shared goal of AI transformation while addressing earlier hurdles like talent gaps, ethical considerations, and technological limitations.
Change Management Strategies
To successfully implement AI, a structured approach to change management is essential. Leaders must cultivate an environment where teams feel both supported and prepared to collaborate with AI tools. This means rolling out well-designed training programs and maintaining open communication to address any concerns or resistance head-on.
Impact Assessment and Planning
A thoughtful, strategic approach is critical for identifying how and where AI can make the biggest difference within an organization. Careful analysis of operations can help:
- Pinpoint areas with high potential for AI integration
- Allocate resources effectively
- Establish realistic timelines
- Define metrics to track success
Training and Support Framework
Training employees is a cornerstone of effective AI adoption. Below is a breakdown of key training components and their goals:
Component | Purpose | Expected Outcome |
---|---|---|
Technical Training | Build hands-on expertise | Confident system usage |
Process Integration | Align AI with workflows | Smooth implementation |
Ongoing Support | Address ongoing challenges | Sustained engagement |
Performance Monitoring | Track progress | Tangible improvements |
Fostering Collaboration
For AI to reach its full potential, leaders need to foster collaboration between human employees and AI systems. This involves showcasing how AI can complement human skills, empowering teams to see it as a tool that enhances their capabilities rather than a replacement.
Documentation and Knowledge Management
Clear documentation is crucial for long-term success with AI. This includes:
- Detailed descriptions of system capabilities and limitations
- Standard operating procedures
- Troubleshooting guides
- Best practices and real-world use cases
Strategic Implementation
Organizations that approach AI adoption methodically often achieve better outcomes. This involves thoroughly analyzing operations, identifying opportunities for AI, and crafting tailored strategies to address specific needs and goals.
5. Meeting AI Regulations and Standards
By 2025, AI leaders are expected to tackle a dense web of regulations, adding to earlier challenges like talent shortages and integration hurdles. Staying competitive while meeting these evolving standards demands a clear understanding of key compliance areas. Here's a snapshot of the primary domains influencing AI operations:
Compliance Area | Requirements | Impact on Operations |
---|---|---|
Data Privacy | Compliance with GDPR and CCPA | Stricter data handling protocols |
Ethics & Fairness | Regulatory audit requirements | Ongoing compliance monitoring |
Transparency | Comprehensive model documentation | Longer development timelines |
Security | Strong access controls and encryption | Increased infrastructure expenses |
Documentation Requirements
To meet regulatory demands, organizations must maintain detailed records, including:
- Sources of data and validation processes
- Model architecture and technical specifications
- Performance metrics and testing outcomes
- Risk management strategies
- Mechanisms for human oversight
Financial Implications
Falling short of compliance can lead to hefty fines and a cascade of indirect costs. These include legal fees, operational disruptions, and damage to reputation. A tarnished reputation can drive away customers, impact market valuation, and make it harder to retain top talent.
Cross-Border Considerations
As AI systems become more integrated globally, navigating differing international regulations adds another layer of complexity. For instance, the United States has introduced initiatives aimed at maintaining its edge in AI innovation. These include partnerships designed to train 2.5 million Americans in fundamental AI skills by 2025.
Governance Structure
A solid governance framework is critical for managing compliance. A three-tiered approach can provide the necessary oversight:
-
Board-Level Oversight
An AI ethics committee, comprising experts in law, technology, and business, offers strategic guidance and reviews high-risk projects. -
Operational Management
Teams on the ground ensure daily compliance while driving business objectives forward. -
Independent Assessment
Regular third-party audits assess the effectiveness of governance and identify areas for improvement.
This structure not only helps organizations meet regulatory demands but also strengthens internal processes.
Trust and Safety Concerns
Roughly 50% of employees express concerns about the accuracy of AI systems. Addressing these worries requires robust validation processes and clear communication to reassure stakeholders about the reliability of AI tools.
Proactive Compliance Strategy
Organizations that excel in this regulatory environment often adopt a "compliance by design" philosophy. By embedding regulatory considerations into the earliest stages of development, they can better anticipate challenges. Experts emphasize that regulatory foresight will play a pivotal role in shaping the future of AI in the United States. This forward-thinking approach is essential for fostering innovation while maintaining a competitive advantage.
Solutions to AI Leadership Challenges
Tackling leadership challenges in AI requires a thoughtful combination of technical know-how and effective change management. It starts with a detailed operational analysis to pinpoint opportunities where AI can have the most impact. From there, leaders can develop a clear roadmap that balances immediate priorities with plans for long-term growth.
Technology Implementation Framework
A well-structured framework is crucial for successful AI adoption. Here's an overview of the key phases:
Phase | Key Activities | Expected Outcomes |
---|---|---|
Assessment | Analyze operations, identify AI opportunities | A clear, actionable AI roadmap |
Development | Design, test, and validate solutions | AI systems tailored to specific needs |
Integration | Deploy systems, optimize workflows | Smooth integration with existing processes |
Maintenance | Monitor and refine performance | Sustained efficiency and effectiveness |
This phased approach ensures smoother implementation, empowers teams, and fosters continuous improvement.
Staff Empowerment and Training
AI systems are only as effective as the teams managing them. Comprehensive training programs equip employees to handle AI tools confidently and extract their full potential. By investing in skill development, organizations give their teams the tools they need to adapt and thrive alongside advancing AI systems.
Continuous Optimization Approach
To keep AI systems performing at their best, organizations must adopt a mindset of ongoing refinement. Here's how:
- Regular Performance Checks: Conduct audits to evaluate accuracy, efficiency, and compliance with regulations. This ensures systems stay aligned with organizational goals.
- Flexible Management: Use performance data to make adjustments as needs evolve. For some, treating AI as a Managed Service (AIaaS) can streamline this process.
- Comprehensive Documentation: Maintain thorough records of technical specs, performance metrics, training guides, and compliance documentation. This supports transparency and simplifies future updates.
Expert Support Integration
For many organizations, navigating the complexities of AI requires external expertise. NAITIVE's advisory services, for instance, have helped businesses align AI solutions with their strategic goals. As one CEO put it:
"The insights gained from NAITIVE AI advisory sessions have been enlightening".
These expert consultations can demystify technical challenges and ensure solutions are both practical and aligned with business objectives.
Risk Mitigation Strategy
To minimize potential pitfalls, implement rigorous testing protocols. This includes evaluating security, system performance, regulatory compliance, and user experience. Catching issues early in the process reduces risks and ensures smoother rollouts.
Conclusion
The rise of AI leadership brings both exciting opportunities and pressing challenges. For organizations adopting AI technologies, the key to success lies in blending technical expertise with forward-thinking leadership.
"Everything changes with AI, this is a time of strategic decisions. Those who adapt and adopt first, win. The 'wait and see' approach is no longer an option."
Real-world success stories show how a thoughtful approach to AI can turn hurdles into avenues for growth and progress. To achieve this, organizations should focus on a few essential strategies:
- Strategic Implementation: Evaluate operations to uncover areas where AI can make the most impact.
- Technical Excellence: Ensure AI integrates smoothly with current systems and processes.
- Team Empowerment: Offer specialized training and resources to help teams adapt effectively.
- Compliance Management: Stay aligned with shifting regulations to avoid setbacks.
FAQs
What are some effective strategies for addressing the AI talent shortage in a rapidly evolving industry?
Addressing the shortage of AI talent calls for a mix of strategic planning and creative solutions. One effective step is investing in the development of current employees. By offering training programs focused on AI tools and technologies, companies not only enhance their internal knowledge base but also strengthen employee commitment and satisfaction.
Collaborating with universities and research institutions is another powerful way to tap into emerging talent. Initiatives like internships, scholarships, and joint research projects can help businesses connect with skilled professionals at the start of their careers. For immediate expertise, companies can also turn to AI consulting services, such as those provided by NAITIVE AI Consulting Agency. These services can help organizations implement AI solutions while addressing talent shortages.
Finally, embracing remote work options or hiring internationally can significantly broaden the talent pool. By being open to global talent and flexible work arrangements, companies can access a diverse range of skilled professionals to meet their AI needs. This adaptable hiring approach can be a game-changer in overcoming the talent gap.
How can companies ensure their AI systems are ethical and free from bias?
To build AI systems that are fair and ethical, companies can take a few key actions:
- Use Diverse Data and Regular Testing: Ensure datasets represent a wide range of demographics and perspectives. Regularly test AI models to uncover and address any biases that might emerge.
- Promote Transparency: Provide clear documentation about how AI models are developed, trained, and how they make decisions. This openness helps maintain accountability.
- Set Ethical Standards: Develop and enforce ethical guidelines for AI projects. Equip teams with proper training to follow these principles throughout the development process.
By focusing on these steps, businesses can build trust and ensure their AI solutions reflect ethical values.
How can AI leaders ensure compliance with regulations like GDPR and CCPA while driving innovation?
AI leaders can successfully navigate the fine line between regulatory compliance and forward-thinking innovation by taking a thoughtful, proactive approach. The first step? Build a culture centered on compliance. Make sure every team member understands the weight of privacy laws like GDPR and CCPA. Regular training sessions and well-defined policies can keep compliance top of mind across the organization.
When developing AI solutions, embed privacy-by-design principles right into the system's foundation. This means weaving data protection measures into the architecture from the start, reducing the risk of compliance issues down the line. You can also use AI tools to simplify compliance tasks - like tracking data usage or flagging potential violations in real time.
Don’t stop there. Keep a close eye on changing regulations and work hand-in-hand with both legal and technical experts. This ensures your AI strategies stay legally sound without stifling innovation. If the landscape feels overwhelming, consider teaming up with consulting agencies that specialize in these areas - they can provide tailored advice to help you navigate this tricky terrain.