How to Calculate AI's Impact on Revenue
Learn how to effectively measure the financial impact of AI on revenue through key metrics, tools, and strategies for businesses.
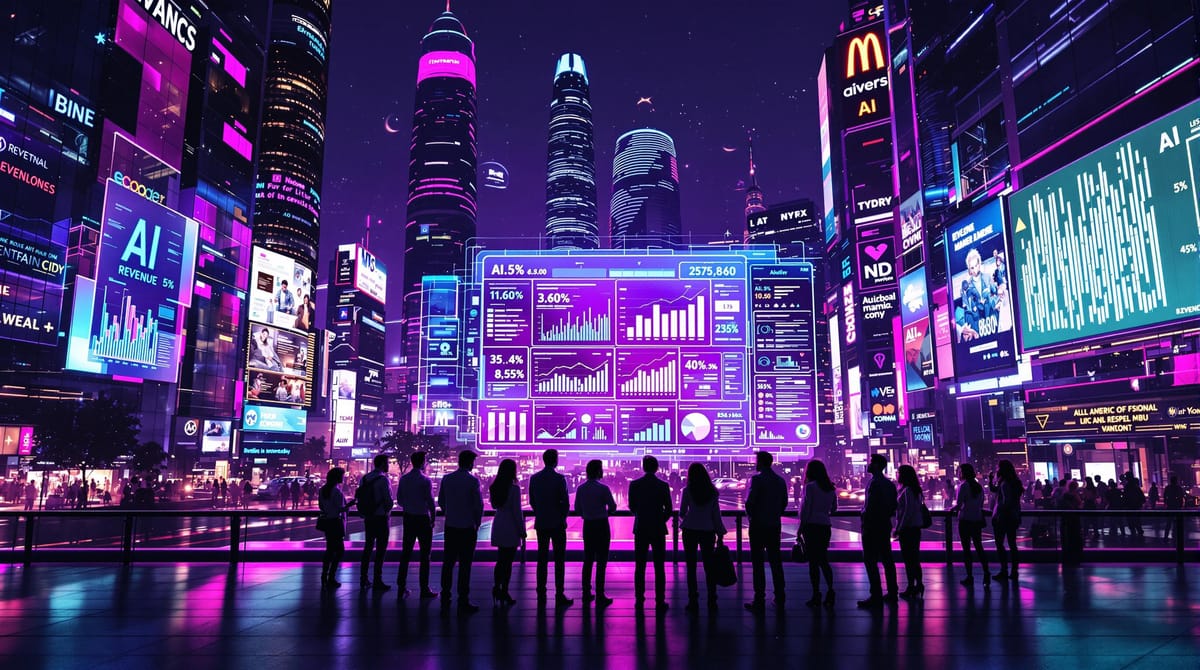
AI can boost revenue by automating tasks, improving decisions, and enhancing customer experiences. But how do you measure its financial impact? Here’s a simple breakdown:
- Why Measure AI's Impact? Justify AI investments and shape strategies. Example: A company saved $120,000 annually using AI for customer inquiries.
- Challenges: Poor data, complex attribution, and delayed results. Solutions include A/B testing, clear baselines, and ongoing assessments.
- Key Metrics: Track Sales Growth, Cost Savings, and Customer Lifetime Value.
- Tools: Use platforms like Tableau for trends, TensorFlow for performance, and Apache Kafka for data integration.
- Methods: Combine A/B testing, revenue attribution, and pre-AI benchmarks to isolate AI’s contributions.
Quick Comparison: Challenges and Solutions
Challenge | Impact | Solution |
---|---|---|
Data Quality Issues | $12.9M annual loss from bad data | Data cleaning and validation |
Attribution Complexity | Hard to isolate AI's role | A/B testing, clear baselines |
Time Discrepancies | Delayed benefits | Ongoing ROI assessments |
AI can directly and indirectly influence revenue. For example, AI chatbots reduce costs by 40-60%, while personalized marketing boosts revenue per customer by 15-25%. Learn how to track these gains effectively.
Measuring AI ROI: Metrics That Matter
Key Metrics for AI Revenue Tracking
Tracking the right metrics and setting clear benchmarks is essential for understanding AI's financial impact. According to McKinsey, generative AI alone could contribute between $2.6 trillion and $4.4 trillion to global corporate profits every year. For businesses adopting AI, this makes precise monitoring a must.
Choosing Revenue KPIs
Focus on measurable KPIs that align with your business goals. Here are some key metrics based on their areas of impact:
Impact Area | Key Performance Indicators | Focus Area |
---|---|---|
Direct Revenue | - Sales Revenue Growth - Customer Lifetime Value - Average Transaction Value |
Tracks immediate financial benefits from AI |
Operational Efficiency | - Cost Reduction - Process Time Savings - Resource Utilization |
Measures savings and productivity gains |
Customer Metrics | - Customer Acquisition Cost - Retention Rate - Satisfaction Scores |
Examines customer-related revenue impacts |
For example, companies in retail and consumer goods using AI have identified potential gains of $400 billion to $660 billion in additional operating profits annually. This highlights the need to monitor both direct revenue growth and cost savings.
Establishing Pre-AI Performance Benchmarks
Setting accurate baseline metrics before introducing AI is key to understanding its real impact. Clear benchmarks help separate AI's contribution from other factors. Here’s how to set them up:
1. Gather Historical Data
Collect at least 12 months of historical data to account for seasonal trends. Focus on metrics such as:
- Monthly revenue
- Customer acquisition costs
- Efficiency metrics
- Customer satisfaction scores
2. Ensure Data Quality
Avoid errors by validating your data. This includes:
- Consistent data collection methods
- Accuracy checks through audits
- Proper documentation of data sources
- Strong governance policies
3. Document Benchmarks
Record all pre-AI metrics thoroughly. Include:
- Methodologies used
- External factors influencing results
- Trends and error margins
With clear KPIs and solid benchmarks in place, businesses can move forward with confidence, ensuring they measure AI's impact effectively.
Data Collection Methods for Revenue Analysis
AI analytics platforms simplify performance tracking, leading to more precise revenue attribution. Collecting accurate data is essential for tracking performance and measuring AI's financial impact effectively.
Tools for AI Performance Tracking
Using the right tools is key to monitoring AI performance effectively. Here's how various analytics tools cater to specific needs:
Tool Category | Key Features | Best Use Case |
---|---|---|
Business Intelligence | Data visualization, custom dashboards, automated reporting | Tools like Tableau and Power BI for analyzing revenue trends |
AI-Specific Analytics | Model performance tracking, learning curves, dataset versioning | TensorFlow and PyTorch for tracking technical metrics |
Integration Tools | API connectivity, real-time data streaming, cross-platform sync | Apache Kafka for seamless data collection across platforms |
Data Quality Standards
Maintaining high data quality is non-negotiable. Key standards include:
Data Validation Framework
- Ensures input data is consistent, complete, and accurate
- Includes automated audits, error detection, and cleaning processes
Security and Privacy Controls
- Implements data encryption
- Enforces strict access controls
- Monitors compliance and maintains audit trails
Apache Beam is a powerful tool for upholding these standards. It supports data validation, ensuring accuracy and consistency across all collection points.
Measuring AI-Specific Revenue Impact
Measuring how AI directly impacts revenue means separating its effects from other business influences. McKinsey research highlights that generative AI alone could contribute $2.6 trillion to $4.4 trillion in global productivity gains, underscoring the importance of precise measurement.
A/B Testing AI Systems
A/B testing is a practical way to evaluate AI's revenue impact. By comparing AI-enhanced processes with traditional approaches, businesses can clearly see the difference. For example, AI has been shown to improve conversion rates by 20-30%, reduce costs by 40-60%, and boost revenue per customer by 15-25%.
Business Area | AI Implementation | Measurement Approach | Typical Impact |
---|---|---|---|
Sales | Predictive Lead Scoring | Compare conversion rates for AI-scored vs. manual leads | 20-30% higher conversion rate |
Customer Service | AI Chatbots | Track resolution times and customer satisfaction | 40-60% cost reduction |
Marketing | AI-Driven Personalization | Compare engagement rates for AI-led vs. standard campaigns | 15-25% increased revenue per customer |
While A/B testing highlights AI's direct effects, broader frameworks like revenue attribution provide a more comprehensive view of its influence across the entire customer journey.
Revenue Attribution Methods
To link revenue growth directly to AI, businesses need detailed attribution methods that track AI's role at every customer interaction. This includes examining cost savings, revenue gains from AI-optimized interactions, and efficiency improvements turned into financial value.
Take Microsoft's Copilot study as an example: users completed tasks up to 73% faster, cutting down routine workloads and positively affecting profit margins through improved productivity. These efficiency gains often translate into major cost savings and revenue growth.
To measure AI's revenue contribution, companies should focus on pre- and post-implementation KPIs, identify AI's specific contributions, and calculate the monetary benefits of efficiency improvements. This approach allows businesses to better understand and quantify AI's impact on their bottom line.
Computing AI Revenue Results
Once businesses have set up measurement frameworks, they can evaluate AI's financial impact by examining both direct and indirect revenue contributions.
Direct Revenue Calculations
Direct revenue focuses on tangible financial gains directly tied to AI systems. Here's a structured approach for calculating these impacts:
Revenue Source | Calculation Method | Example Metric |
---|---|---|
Sales Increase | Post-AI sales - Pre-AI sales | 15% boost in e-commerce sales from AI recommendations |
Cost Reduction | Previous costs - AI-enabled costs | 40% cut in operational expenses |
Time Savings | Hours saved × hourly rate | $360 saved per mortgage application review |
Indirect Revenue Effects
Indirect revenue contributes long-term value through strategic improvements. These include smoother operations, better customer experiences, and faster innovation. For instance, Waggel, a pet insurance provider, adopted an AI data platform, cutting processing times by 35% and increasing customer retention by 28%. This showcases how operational efficiency directly impacts business outcomes.
"Measuring AI ROI requires shifting focus from immediate financial results to broader strategic value."
Key areas to track include:
- Operational efficiency: Measure reductions in processing times and resource usage.
- Customer satisfaction: Observe improvements in retention rates and lifetime customer value.
- Innovation: Track the speed of new product launches and success in entering new markets.
By blending these direct and indirect insights, businesses can present a clear picture of AI's financial impact to stakeholders.
Creating Revenue Reports
To effectively communicate AI's value, reports should combine measurable outcomes with strategic benefits. For example, OTTera used advanced analytics to expand its market reach, becoming one of the fastest-growing PaaS providers.
Report Component | Key Metrics | Data Sources |
---|---|---|
Direct Impact | Revenue growth, cost savings | Financial systems, sales data |
Indirect Benefits | Efficiency, customer satisfaction | Operational metrics, surveys |
Conclusion: Next Steps with AI Revenue Data
Key Steps to Measure AI's Revenue Impact
Understanding AI's impact on revenue requires a well-structured plan that evaluates both immediate outcomes and long-term benefits. Start by setting clear performance benchmarks and gathering reliable baseline data. From there, implement tools to track both direct financial results and less obvious advantages.
Here's a simplified framework for measuring AI's impact:
Phase | Key Activities | Success Factors |
---|---|---|
Initial Assessment | Collect baseline data, define KPIs | Clear objectives and data quality |
Implementation | Conduct A/B tests, monitor performance | Consistent tracking methods |
Analysis | Calculate direct and indirect impacts | Broad evaluation perspective |
Optimization | Refine strategies, adjust systems | Focus on ongoing improvements |
For instance, a healthcare study in 2024 revealed a 451% return on investment from AI over five years, showcasing the importance of thorough measurement practices.
NAITIVE AI Consulting Agency: Your Partner in AI Success
If you're looking for expert help to navigate these steps, a professional consulting agency can make all the difference. NAITIVE AI Consulting Agency specializes in crafting tailored AI solutions that deliver measurable results, especially in areas like autonomous agents and process automation.
Their expertise can help your organization:
- Regularly evaluate and adjust AI strategies based on performance data
- Maintain high standards for data quality
- Incorporate AI metrics into broader business reviews to scale successful initiatives
FAQs
Here are clear answers to some common questions about evaluating AI's financial impact, based on the concepts covered in this guide.
How do you measure ROI from AI?
To calculate ROI from AI, use this formula: (Net Benefits ÷ Total Costs) × 100. Net benefits include factors like increased revenue, cost reductions, and improved efficiency. Total costs account for implementation, maintenance, and infrastructure. For example, AI-powered inventory management might reduce overstock by 15%, boost sales by 10%, and cut storage expenses. Regularly update these figures to reflect ongoing AI performance.
How to calculate ROI for an AI project?
As discussed in the 'Key Metrics' and 'Direct Revenue Calculations' sections, ROI calculations should factor in both measurable outcomes and broader business benefits. Key considerations include:
- Initial implementation costs
- Ongoing operational expenses
- Revenue growth
- Efficiency improvements
- Changes in workforce productivity
Set clear KPIs before starting the project and track progress consistently to ensure accurate ROI measurement.
How do you measure the impact of AI in business?
Referring to the 'Measuring AI-Specific Revenue Impact' section, you can evaluate AI's contribution to your business by monitoring these key metrics:
- Conversion rates: Check for improvements in customer conversions after AI implementation.
- Customer behavior: Track changes in repeat purchase rates or other engagement metrics.
- Revenue trends: Compare revenue before and after introducing AI.
Reliable results depend on using high-quality data and maintaining consistent tracking. Regularly reviewing and fine-tuning AI systems helps maximize their performance and measure both direct effects (like higher sales) and indirect benefits (such as better customer satisfaction and streamlined operations).