Exploring the Future of Generative AI with NVIDIA NIM™ Agent Blueprints
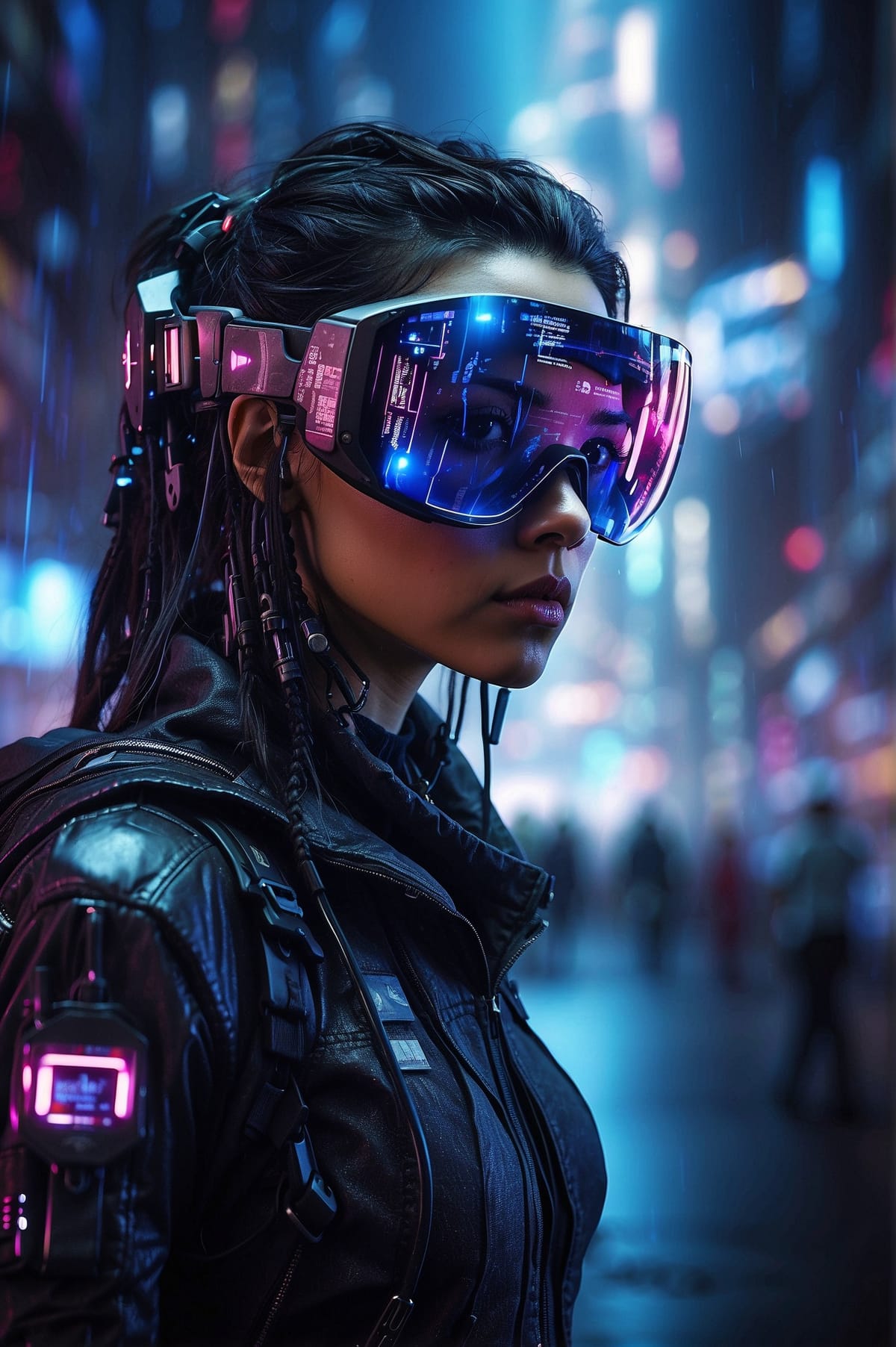
TL;DR
Generative AI’s future is full of potential, with market projections of $190 billion by 2025. Businesses should prepare by fostering technological adaptability and ethical AI deployment, focusing on transparency, data security, and stakeholder trust. The journey ahead promises transformative advancements and significant industry shifts.
Understanding NVIDIA NIM™ Agent Blueprints
In a significant technological advancement, NVIDIA recently unveiled its NIM™ Agent Blueprints. These innovative blueprints are essentially reference applications designed for canonical generative AI use cases. Each blueprint encompasses a variety of components, including NVIDIA NIM and partner microservices, one or more AI agents, open-source sample code, detailed customization instructions, and even a Helm chart for deployment.
What are the NIM™ Agent Blueprints?
NIM™ Agent Blueprints represent a comprehensive framework for developers, providing a structured and guided approach to implementing generative AI technologies. These blueprints simplify complex AI development processes, allowing developers to focus more on creativity and engineering rather than getting bogged down by technical intricacies. As an overall package, each blueprint is designed to be a versatile and adaptable solution, making it ideal for a wide range of applications, from enterprise-level deployments to innovative startup solutions.
The remarkable aspect of these blueprints lies in their capability to be customized and upgraded according to specific business needs. Whether an organization is looking to enhance its customer service with AI-powered digital avatars or aims to explore drug discovery through generative virtual screening, NVIDIA NIM™ Agent Blueprints offer a robust foundational canvas tailored for diverse objectives.
Core Components and Technologies Involved
Delving into the core components, each NIM™ Agent Blueprint includes a variety of sophisticated elements. Notably, these blueprints incorporate pre-built microservices from NVIDIA and its partners that function seamlessly with AI agents. The open-source sample code provides a solid groundwork for developers to extend and refine the functionalities as per their project requirements.
Overall, the inclusion of Helm charts paves the way for simplified deployment processes. Helm charts are instrumental in managing Kubernetes applications, significantly streamlining the process of deploying AI solutions in cloud or on-premises infrastructures. This level of deployment flexibility is crucial for businesses aiming to leverage AI across different environments.
- Generative Virtual Screening for Drug Discovery: This use case focuses on sifting through and optimizing a library of small molecules to pinpoint chemical structures that can bind to a target protein. This blueprint can revolutionize how pharmaceutical companies approach drug discovery.
- Digital Humans for Customer Service: This approach involves using AI-powered digital avatars to enhance customer service experiences. Businesses can deploy highly interactive, lifelike avatars as a more engaging customer service interface.
- Multimodal PDF Data Extraction for Enterprise (RAG): Utilizing NVIDIA NeMo™ Retriever microservices, this blueprint helps enterprises unlock accurate insights from massive volumes of data contained within PDFs, making it a powerful tool for data-driven decision-making.
Potential for Customization and Deployment
One of the standout characteristics of the NIM™ Agent Blueprints is their exceptional versatility in terms of customization and deployment. Developers can modify these blueprints by integrating proprietary data, thereby creating AI models that are finely tuned to meet their unique requirements.
Furthermore, the blueprints are designed to be easily deployable across various infrastructures — be it in a traditional data center or a modern cloud environment. This level of adaptability ensures that both startups with limited resources and established enterprises with complex IT environments can adopt these blueprints effortlessly.
NVIDIA’s innovation in this space demonstrates the company’s commitment to making advanced AI technologies accessible and practical. The versatility of these blueprints is clearly evidenced by the skyrocketing growth of AI technology use cases, as seen by the 42% increase in AI implementations across various industries in 2023. These blueprints are not merely tools but enablers that empower organizations to dream bigger and build smarter solutions.
As AI continues to revolutionize multiple sectors, from healthcare to finance, the adaptability and robust customization features of NVIDIA NIM™ Agent Blueprints will likely play a crucial role in shaping the future landscape of AI-driven innovations.
“NVIDIA’s innovation in AI technology allows us to dream bigger and build smarter solutions for tomorrow’s challenges.” — AI Expert
Indeed, in today’s fast-evolving tech ecosystem, having a structured yet flexible approach to AI deployment is invaluable. The NIM™ Agent Blueprints offer a glimpse into a future where AI solutions are not only more accessible but also highly customizable to meet the ever-changing demands of businesses and industries worldwide.
Key Applications of NVIDIA NIM™ in Industry
Transformative changes are driven by the integration of cutting-edge technologies in various industries. NVIDIA NIM™ is one such advancement, presenting powerful AI solutions through its Agent Blueprints. These blueprints encompass an array of applications, including generative virtual screening for drug discovery, digital humans in customer service, and multimodal PDF data extraction for enterprises. Each of these applications is poised to revolutionize its respective field with unprecedented efficiency and accuracy.
Generative Virtual Screening for Drug Discovery
Generative virtual screening is a game-changer in the realm of drug discovery. This technique leverages artificial intelligence to sift through extensive libraries of molecules at a speed and accuracy previously unimaginable. Traditionally, drug discovery has been a time-consuming and resource-intensive process. However, with AI-powered generative virtual screening, researchers can now identify potential drug candidates rapidly, expediting the journey from concept to clinical trials.
At the heart of this process is the ability of AI to predict molecular behavior and interactions. Utilizing NVIDIA NIM™, researchers can optimize a library of small molecules to pinpoint chemical structures that have a high likelihood of binding to a specific target protein. This approach not only accelerates the discovery phase but also enhances the precision and success rate of identifying viable drug candidates.
Consider a hypothetical scenario where researchers are searching for a treatment for a new viral disease. Employing generative virtual screening, they input the molecular structure of the virus’s protein into the AI system. The AI then analyzes millions of molecular compounds, ranking them based on their potential efficacy. This streamlined process not only saves valuable time but also opens up new possibilities for rapid response to emerging health threats.
Digital Humans in Customer Service
The landscape of customer service is undergoing a seismic shift with the introduction of digital humans. These AI-powered avatars are capable of simulating human interactions with remarkable realism. By integrating digital avatars into customer service platforms, businesses can enhance the quality of customer interactions, offering personalized and efficient support around the clock.
A digital human, equipped with NVIDIA NIM™, can understand and respond to customer queries in real-time. This capability not only reduces wait times but also delivers a more consistent and satisfying user experience. The digital avatar can perform tasks ranging from answering frequently asked questions to resolving complex issues, all while maintaining a friendly and engaging demeanor.
“The digitization of customer service through AI avatars is not just a trend, it is a transformation in user engagement.” — Industry Leader
Moreover, as digital avatars gather data from customer interactions, they become increasingly adept at personalizing responses. This continuous learning loop ensures that the service provided becomes progressively more tailored to individual customer needs. Businesses across various sectors, such as retail, banking, and telecommunications, are recognizing the potential of AI avatars to revolutionize their customer service frameworks.
Multimodal PDF Data Extraction for Enterprises
The vast repositories of data stored in multimodal PDF documents pose a significant challenge for enterprises when it comes to efficient data extraction and analysis. Traditional methods of manual data entry and processing are not only labor-intensive but also prone to errors. NVIDIA NIM™ offers a groundbreaking solution with its multimodal PDF data extraction capabilities, transforming how businesses handle large volumes of data.
By employing NVIDIA NeMo™ Retriever NIM microservices, enterprises can automate the extraction of structured data from PDF documents. This process integrates various data types, including text, images, and tables, providing comprehensive insights with minimal human intervention. The AI system is capable of understanding context and relevance, thereby ensuring that the extracted data is not just accurate but also meaningful.
Imagine a financial institution that needs to process thousands of loan applications stored in PDF format. Using traditional methods, this task would require significant manpower and time, not to mention the potential for human error. However, with multimodal data extraction powered by NVIDIA NIM™, the institution can automate the extraction of key information such as applicant details, financial history, and risk assessments. This not only speeds up the processing time but also enhances the accuracy and reliability of the data.
- NVIDIA NeMo™ Retriever NIM microservices are crucial for parsing and analyzing multimodal data.
- Automated data extraction significantly reduces the burden of manual processing.
- Enterprises can leverage AI to gain actionable insights from vast datasets.
These applications collectively illustrate the broader impact of NVIDIA NIM™ in various industries. From accelerating drug discovery to redefining customer service and automating document processing, NVIDIA NIM™ is at the forefront of creating intelligent, efficient, and scalable AI solutions.
Furthermore, according to recent data, 85% of businesses consider AI to significantly enhance customer experience by 2025. This statistic underscores the growing recognition of AI’s potential and the readiness of industries to adopt advanced technologies such as NVIDIA NIM™.
As industries embrace these innovations, the role of AI in driving efficiency, precision, and customer satisfaction becomes ever more evident. Organizations looking to stay ahead in a competitive landscape will find invaluable advantages in integrating NVIDIA NIM™ into their operational workflows.
Innovative AI Models Offered by NVIDIA
NVIDIA has continually advanced the technology landscape with its groundbreaking AI models, offering unparalleled capabilities across various domains. This section delves into some of NVIDIA’s most innovative models, such as Llama-3 and AlphaFold2, outlining their applications, benefits, and the advantages of deploying them in self-hosted environments.
Introduction to Various Models Like Llama-3 and AlphaFold2
Among NVIDIA’s impressive lineup of AI models, the Llama-3 series stands out for its specialization in language capabilities. The Llama-3-Swallow 70B model, for example, has been tailored specifically for the Japanese language, making it highly efficient in understanding and generating Japanese text. This precision in linguistic nuances is pivotal for enhancing user interaction and engagement.
On the other hand, AlphaFold2, developed by DeepMind and integrated into NVIDIA’s ecosystem, has revolutionized the field of structural biology. It accurately predicts 3D protein structures from amino acid sequences, a feat that has significantly advanced scientific research and medical innovation. According to a protein researcher, “The ability to accurately predict protein structures has opened new avenues for biological research.”
Use Cases of Each Model Across Different Fields
The application of these models spans various industries and use cases. For example, Llama-3’s high proficiency in Japanese can be leveraged in customer service chatbots, translations, and content creation specifically catered to Japanese-speaking audiences. This specialization ensures that communication is not only accurate but also culturally relevant.
AlphaFold2’s contributions to the scientific community cannot be overstated. It helps in drug discovery by allowing researchers to understand protein structures better, thereby identifying potential drug targets more efficiently. This model has been a game-changer in genomics, making precise protein predictions a mainstream capability.
An intriguing use case highlighted by NVIDIA is the Generative Virtual Screening for Drug Discovery, wherein AI searches and optimizes a library of small molecules to identify those that bind effectively to a target protein. Such applications showcase how generative AI is paving the way for breakthroughs in medicinal chemistry.
Advantages of Using These Models in Self-Hosted Environments
One of the critical advantages of NVIDIA’s AI offerings is the flexibility they provide in deployment. Self-hosting these models can be incredibly beneficial for organizations looking to maintain control over their data while still leveraging state-of-the-art AI capabilities.
- Data Security: Companies can ensure that sensitive data remains on-premises, adhering to strict compliance and security policies.
- Customization: Organizations have the freedom to customize these models to better align with their specific needs and business objectives.
- Performance Optimization: With the ability to use NVIDIA-accelerated infrastructure, computing power can be maximized to achieve faster processing times and higher throughput.
For instance, deploying the Llama-3-Swallow 70B on local servers can significantly boost the efficiency and accuracy of Japanese language processing without the concerns over data privacy that come with third-party hosting solutions. Similarly, researchers using AlphaFold2 in a self-hosted environment can benefit from immediate access to computation results, enabling quicker iterations in their experiments.
Additional Available Models
NVIDIA also offers other models for exploration through a browser or with free credits, making it accessible for developers and researchers to experiment and innovate. Notable examples include:
- ChatGLM: Supports both Chinese and English languages, handling tasks like chatbots, content generation, coding, and translation.
- Florence-2 0.7B: A vision foundation model that excels in computer vision and vision-language tasks.
- Jamba 1.5: An advanced mixture-of-experts (MOE)-based model designed for varied generative AI tasks.
- Mistral-NeMo-Minitron-8B: A highly accurate distilled model suitable for chatbots, virtual assistants, coding, and content generation.
By providing such a diverse arsenal of AI tools, NVIDIA ensures that developers and enterprises have the resources they need to drive innovation and solve complex challenges across numerous fields. The flexibility to self-host these models further empowers organizations to innovate rapidly while maintaining robust data governance and customization capabilities.
The Future of Generative AI & Its Implications
Generative AI is set to revolutionize the technological landscape over the next decade, bringing forth unprecedented possibilities and challenges. As artificial intelligence continues to advance at breakneck speed, understanding its future implications becomes crucial for businesses looking to stay ahead of the curve. This section delves into expectations for AI in the coming years, strategies for integrating AI into business operations, and the ethical considerations that must be addressed.
Expectations for AI in the Next Decade
AI innovation is growing at an astronomical rate, with predictions suggesting the AI market will hit a staggering $190 billion by 2025. Such growth underscores the transformative potential that AI holds across various sectors. In the next ten years, expectations include more sophisticated AI models capable of performing complex tasks and generating human-like content with remarkable accuracy. Additionally, AI is anticipated to enhance personalization in customer service, drive efficiencies in manufacturing, and revolutionize areas like drug discovery and healthcare.
Notable advancements such as NVIDIA’s introduction of NIM™ Agent Blueprints highlight the practical applications of generative AI. These blueprints cover essential use cases, from generative virtual screening for drug discovery to digital humans for customer service, showcasing how AI can seamlessly blend into existing workflows. Looking forward, generative AI will likely become a cornerstone of multifaceted applications, including autonomous systems and intelligent data extraction tools.
How Businesses Can Prepare for AI Integration
As AI becomes an integral part of business strategy, companies must adopt a proactive approach to successfully integrate these technologies. The first step involves fostering a technologically adaptive culture within the organization. This includes training employees to work alongside AI and promoting continuous learning to stay abreast of AI advancements.
Implementing AI requires robust infrastructure and access to significant data. Businesses need to invest in scalable and flexible IT infrastructure to support AI deployment. Moreover, partnerships with AI providers like NVIDIA can provide tailored solutions that are cost-effective and scalable. NVIDIA’s recently launched inference microservices for models such as Llama-3-Swallow 70B and Florence-2 0.7B exemplify resources that businesses can tap into for a seamless AI integration process.
Additionally, it’s crucial for businesses to pilot AI projects on a smaller scale to gauge their impact before broader rollout. Iterative testing and feedback can help refine AI applications, ensuring they deliver the intended outcomes efficiently.
In summary, the future of generative AI holds immense promise, with market growth projected to reach $190 billion by 2025. For businesses, adapting to technological advancements will be crucial, involving employee training and a robust IT infrastructure. Navigating the ethical landscape will require transparency, data security, and proactive stakeholder engagement.