Data Standardization Challenges in AI Systems
Explore the challenges of data standardization in AI systems, including solutions and future trends for improving performance and compliance.
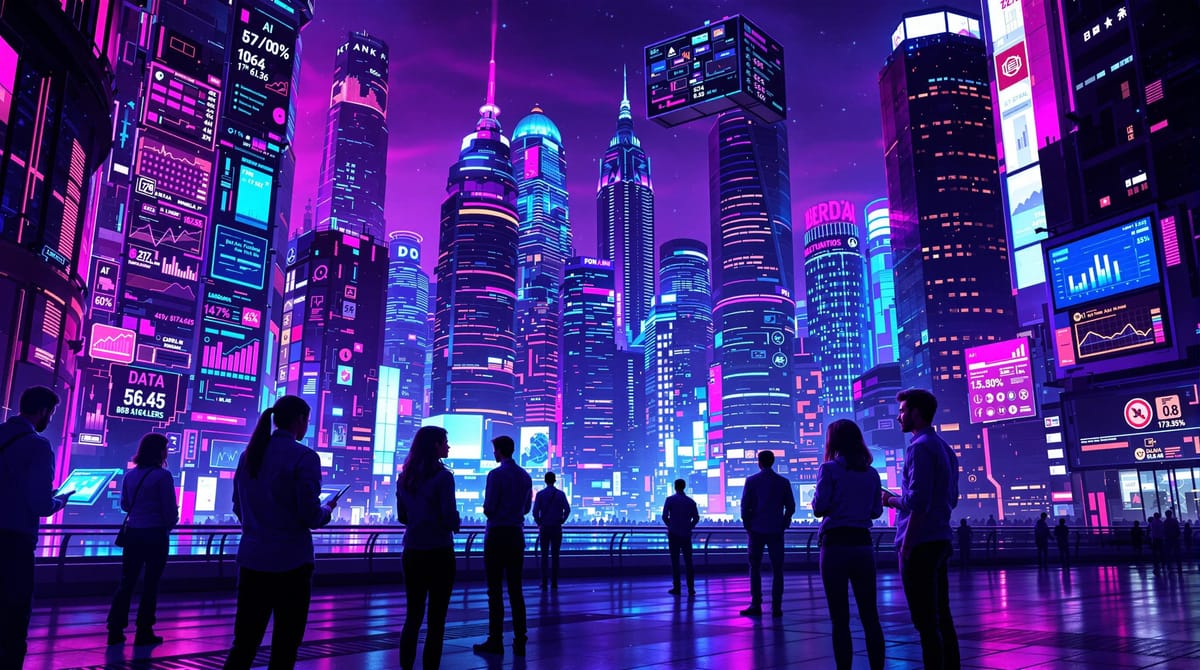
Data standardization is essential for AI systems to function effectively, but it comes with challenges. Here's what you need to know:
- Why it matters: Standardized data improves AI accuracy, reduces costs, and ensures compliance with regulations. Without it, AI systems fail to deliver reliable results.
- Key challenges: Mismatched formats, inconsistent quality, and legacy systems make data standardization difficult.
- Solutions: Use clear governance frameworks, AI-powered tools, and staff training to streamline the process.
- Future trends: Real-time data processing and autonomous AI agents are making standardization faster and more efficient.
Is Your Data “AI-Ready”? Fixing Data Quality Issues
Main Data Standardization Challenges
AI systems often run into hurdles when it comes to standardizing data, which can negatively impact their performance and results. Below are some key challenges that make data standardization difficult.
Issues with Multiple Data Sources
Legacy systems often use inconsistent structures and naming conventions, leading to mapping errors. According to NAITIVE AI Consulting Agency (https://naitive.cloud), aligning schemas is critical to successfully integrating AI systems.
Problems with Data Format and Quality
Inconsistent data formats and varying quality levels create additional complications. For example, differences in date formats, numeric representations, or text encodings can disrupt AI training. Proper data cleansing and normalization are essential for smooth and reliable processing.
Effects on AI System Performance
Poorly standardized data can hurt AI systems in multiple ways - it can lower model accuracy, increase costs, and expose organizations to compliance risks. Here's how these challenges unfold:
Reduced AI Model Accuracy
When data quality is inconsistent, AI models struggle to perform well. For example, if a healthcare AI system encounters mismatched date formats in its training data, it might misread critical timelines. This could lead to errors that impact patient care.
Increased Costs and Delays
Fixing data issues takes time and resources. Teams often need to spend extra hours cleaning and validating data, which can delay projects and drive up costs. Instead of focusing on improving model capabilities, they’re stuck addressing inconsistencies.
Compliance and Legal Risks
Inconsistent data management can lead to violations of data protection laws. This exposes organizations to potential lawsuits and reputational harm.
To address these issues, strategies like strong data governance, advanced AI tools for data management, and thorough staff training are essential. NAITIVE AI Consulting Agency emphasizes these methods to ensure data consistency and better AI performance. Up next, we'll explore practical steps to secure and standardize data for AI systems.
Solutions for Data Standardization
Addressing issues that affect AI performance starts with implementing strong data standardization practices.
Steps for Data Governance
Set up a clear data governance framework with well-defined roles and responsibilities. Focus on:
- Data quality metrics and monitoring: Regularly track the accuracy, completeness, and consistency of data.
- Standard naming conventions: Ensure uniformity in how data elements are labeled.
- Validation rules: Create checks to verify data integrity.
- Change management procedures: Establish protocols for handling updates or modifications to datasets.
- Compliance requirements: Align data practices with relevant regulatory standards.
Incorporate change management processes and periodic audits, such as quarterly reviews, to maintain high data quality and consistency.
AI-Powered Tools for Data Management
Leverage advanced AI tools to simplify and enhance data standardization efforts. These tools can:
- Identify and fix inconsistencies in real time.
- Harmonize data formats across different sources.
- Highlight potential quality issues for further inspection.
- Produce detailed reports on data quality metrics.
By automating these tasks, AI tools work alongside governance measures to ensure data remains consistent and reliable.
Training Programs for Staff
Even with governance and automation in place, well-trained staff are essential for maintaining data standards. Develop comprehensive training programs that include:
- Role-Based Training: Create specific modules for data analysts, business users, and decision-makers to address their unique needs.
- Hands-On Workshops: Use real company data in practical exercises to show how data quality impacts AI performance.
- Ongoing Support: Provide access to a knowledge base with documentation, best practices, and expert advice for continuous learning.
This combination of governance, technology, and skilled personnel ensures a strong foundation for effective data standardization.
Future of Data Standardization
Live Data Processing
Processing live data is crucial for AI systems that need to analyze information instantly. Advanced AI tools help cut down response times while boosting accuracy. For instance, NAITIVE's Voice AI Agent solution handles 200 outbound calls daily, all while keeping data formats consistent and standardized.
Real-time standardization is becoming more streamlined with the help of new technologies.
New Technology Standards
Autonomous AI agents are taking on complicated tasks and maintaining consistent data quality across various channels. Businesses are increasingly using AI for tasks like contact center automation (26%), personalization (23%), customer acquisition (22%), product improvements (22%), and new development efforts (19%).
These advancements are paving the way for better quality control systems.
AI Quality Control
AI-driven quality control systems work alongside governance and automation to identify and fix standardization issues in real time.
"The Voice AI Agent Solution NAITIVE implemented is from the future" – John, CEO
These systems act as 'Employee as a Service,' providing 24/7 monitoring to keep data consistent and reliable, even as the volume and complexity of data grow.
Conclusion
Standardizing data is key to making AI work effectively and minimizing risks. Without high-quality, consistent data, AI systems can’t perform at their best.
To achieve this, organizations need strong governance, automated AI tools, and focused employee training. These steps ensure data remains consistent and reliable.
Maintaining data quality is an ongoing effort. Companies should embrace new AI technologies to tackle standardization challenges. For instance, NAITIVE demonstrates how Generative AI can help systems address these issues automatically. These methods pave the way for long-term success.
Here’s what businesses should focus on:
- Establish clear data governance policies
- Use AI tools for real-time standardization
- Provide continuous training for staff
- Integrate smoothly with current systems
- Regularly monitor and improve data quality