Checklist for AI Cost Forecasting Setup
Learn how to effectively set up AI cost forecasting to enhance budget accuracy, resource management, and risk mitigation for your projects.
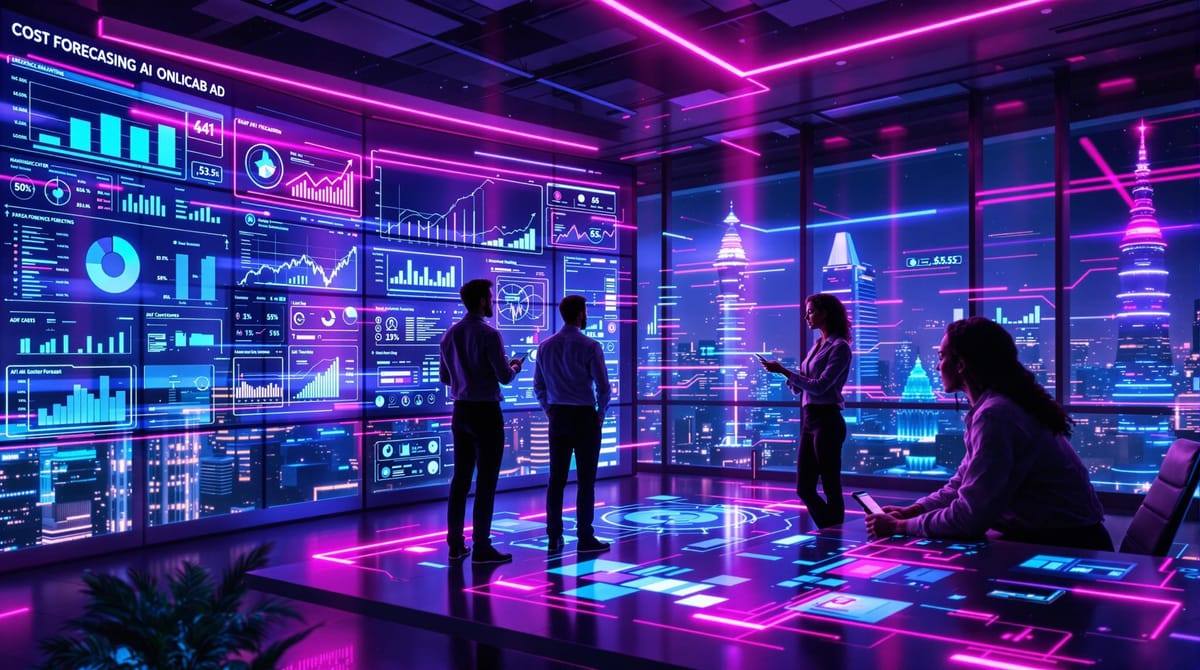
AI cost forecasting helps businesses predict and manage expenses for AI projects, improving budget accuracy, resource allocation, and risk management. Here's a quick guide to set up your system:
-
Set Clear Goals:
- Define business targets (budget limits, KPIs, timelines).
- Identify cost components (infrastructure, software, personnel, training, maintenance).
- Focus on high-impact AI projects like customer acquisition or contact center automation.
-
Build Data Systems:
- Collect reliable data (usage metrics, financial data, performance stats).
- Fix data quality issues (completeness, accuracy, timeliness).
- Integrate data sources (cloud tools, financial systems, project trackers).
-
Pick Tools:
- Choose forecasting software (consider integration, scalability, and security).
- Decide between off-the-shelf or custom-built solutions.
- Create tracking systems for usage, costs, and alerts.
-
Develop Prediction Models:
- Select models (time series, regression, machine learning, hybrid).
- Test accuracy (aim for <15% error).
- Plan for scenarios like growth, cost optimizations, or market disruptions.
-
Integrate and Train:
- Link forecasting tools to finance systems.
- Train staff on system use, data interpretation, and troubleshooting.
-
Monitor and Improve:
- Compare forecasts to actual costs.
- Adjust models and assumptions regularly.
- Maintain data quality and refine predictions.
Why It Matters
Accurate AI cost forecasting ensures smarter investments, better ROI, and fewer surprises. Use this checklist to stay ahead and optimize your AI spending.
Stop Wasting Time on AI Cost Optimization! Focus on This ...
1. Set Clear Goals
Clear goals are the backbone of accurate AI cost forecasting. They guide data collection and tool selection, ensuring every step aligns with your business needs.
1.1 Set Business Targets
Tie cost forecasting to your business objectives to monitor spending and evaluate ROI effectively. Pay attention to these areas:
- Budget Control: Define spending limits for each AI project.
- Performance Metrics: Establish measurable KPIs to track success.
- Timeline Milestones: Schedule checkpoints to review costs and progress.
1.2 List Cost Components
Identify all AI-related expenses to improve the accuracy of your forecasts. Key cost categories include:
Cost Category | Examples of Components |
---|---|
Infrastructure | Cloud services, servers, storage |
Software | Licenses, API usage, custom development |
Personnel | Data scientists, engineers, trainers |
Training | Staff development, certifications |
Maintenance | Updates, optimizations, security measures |
1.3 Select Key AI Projects
Concentrate your AI investments on impactful initiatives such as:
- Contact center automation
- Personalization engines
- Customer acquisition systems
- AI-driven product improvements
Evaluate projects based on factors like scale, resource demands, and complexity of integration. Prioritizing high-impact opportunities that align with your strategic goals ensures your forecasting efforts focus on areas that deliver the most value.
2. Set Up Data Systems
Building a solid data system requires careful planning and execution.
2.1 Check Available Data
Accurate forecasting depends on having reliable and complete data.
Data Category | Required Information | Common Sources |
---|---|---|
Usage Metrics | API calls, compute hours, storage volume | Cloud platform dashboards |
Financial Data | Historical costs, budget allocations, ROI metrics | Accounting systems |
Performance Data | Resource utilization, efficiency metrics | Monitoring tools |
Project Metrics | Development timelines, resource allocation | Project management systems |
This initial review helps pinpoint areas where data quality can be improved.
2.2 Fix Data Quality Issues
The accuracy of your forecasts depends on the quality of your data. Here are key issues to address:
- Completeness: Make sure all cost-related data points are consistently tracked.
- Accuracy: Cross-check data with financial records to eliminate errors.
- Timeliness: Implement real-time data collection whenever possible.
To close data gaps and improve quality:
- Automate data validation to catch errors early.
- Standardize data entry processes across teams.
- Document how data is collected and maintained.
- Schedule regular audits to keep quality in check.
2.3 Connect Data Sources
Bringing together data from different systems is essential for a unified approach.
Key Integration Areas:
- Link cloud monitoring tools with financial systems for a complete picture.
- Connect project management platforms to resource tracking tools.
- Sync vendor billing systems with internal cost tracking mechanisms.
Steps to Implement Integration:
- Map out how data flows between systems.
- Use secure protocols to transfer data safely.
- Automate synchronization to keep information up to date.
- Set up alerts to catch and address errors quickly.
3. Pick and Set Up Tools
Use your integrated data systems to guide the selection and setup of tools for forecasting and tracking.
3.1 Select Forecasting Software
Choose forecasting tools that align with your specific needs. Focus on:
Key Features:
- Data processing capacity
- Integration capabilities
- Customization options
- Scalability
- Security measures
Feature Category | Basic Capabilities | Advanced Options |
---|---|---|
Data Processing | Historical cost analysis, Basic forecasting | Machine learning, Pattern recognition |
Integration | API connectivity, Standard formats | Custom connectors, Real-time updates |
Reporting | Basic cost reports, Usage tracking | Detailed analytics, Custom dashboards |
Security | Data encryption, Access controls | Audit trails, Compliance tools |
3.2 Compare Build vs Buy
Decide whether to build a custom tool or purchase an off-the-shelf solution. This decision impacts both your timeline and budget.
Off-the-Shelf Solutions:
- Quick to implement
- Lower upfront costs
- Regular updates included
- Limited customization
- Predefined features
Custom-Built Solutions:
- Complete control over functionality
- Tailored to unique workflows
- Higher initial costs
- Longer development time
- Requires ongoing maintenance
Consider your available resources, budget, and specific needs. If your organization has unique workflows, a custom solution could offer better long-term benefits despite the higher upfront investment.
3.3 Create Tracking Systems
Robust tracking systems are crucial for accurate cost management.
- Usage Monitoring: Track API calls, compute usage, storage, and model training time.
- Cost Attribution: Assign costs to projects, monitor department-specific usage, and track ROI metrics.
- Alerts: Set up notifications for budget limits, unexpected usage spikes, performance issues, and forecast deviations.
These systems ensure you stay on top of costs and can quickly address any anomalies.
4. Create Prediction Models
Using integrated data systems and effective tracking, your prediction models should reflect established cost drivers.
4.1 Pick Model Types
Choose models that match your data complexity and business objectives. Here are some options:
Model Type | Best For | Requirements |
---|---|---|
Time Series | Regular cost patterns, trends | At least 12 months of consistent data |
Regression | Analyzing multiple cost factors | Clean historical data with identified variables |
Machine Learning | Complex patterns, large datasets | High-quality training data and computing power |
Hybrid | Mixed cost behaviors | Multiple data sources and advanced analytics |
The decision should align with your available data and resources. For instance, if you're dealing with seasonal cloud costs, a time series model works well.
Once you've selected a model, the next step is to test its performance.
4.2 Test Model Accuracy
Validate how well your model performs by using historical data:
- Split your dataset: 70% for training, 30% for testing.
- Perform backtests using known outcomes.
- Measure accuracy with Mean Absolute Percentage Error (MAPE).
- Compare the model's predictions to actual costs.
- Track improvements in prediction accuracy.
Aim for a MAPE below 15% to ensure reliable cost forecasting. Test your model across different timeframes to confirm consistent results.
Once accuracy is confirmed, adapt the model for various business conditions.
4.3 Plan for Different Scenarios
Your models should be flexible enough to handle changing business situations and market conditions.
Key Scenarios to Consider:
- Base Case: Assumes current growth trends.
- Aggressive Growth: Projects 2-3x increases in usage.
- Conservative Case: Accounts for reduced resource use.
- Market Disruption: Prepares for sudden cost changes.
For each scenario, define adjustment factors to quickly update your forecasts.
Scenario Testing Framework:
Scenario Type | Variables to Monitor | Adjustment Triggers |
---|---|---|
Base Case | Standard usage patterns | ±10% variance |
Growth | Resource utilization rates | 25% or more increase |
Conservative | Cost optimization metrics | 15% or more decrease |
Disruption | Market pricing changes | 30% or more variance |
Review scenarios monthly and adjust your models when actual costs deviate beyond the set triggers. This approach ensures your forecasts stay relevant and actionable.
5. Put Forecasting to Work
5.1 Link to Finance Systems
Connecting AI cost forecasting tools with your financial systems streamlines budget management and ensures smoother operations. To make this happen, establish strong data connections between your forecasting tools and finance platforms. Here's what to prioritize:
System Type | Integration Priority | Key Considerations |
---|---|---|
ERP Systems | High | Real-time data sync, automated reconciliation |
Accounting Software | High | Cost categorization mapping, audit trails |
Budgeting Tools | Medium | Forecast vs. actual comparisons |
Business Intelligence | Medium | Custom dashboard creation |
While setting up these connections, focus on proper data validation and error handling to keep your workflows accurate and reliable. Once the systems are integrated, ensure your team is trained to use and manage these tools effectively.
5.2 Train Staff
Training your staff is critical for getting the most out of your forecasting tools. Key areas to cover include:
- Basic System Navigation: Teach day-to-day operations and common tasks.
- Data Interpretation: Help staff understand and analyze forecasting outputs.
- Troubleshooting: Equip them to handle typical system issues.
- Update Procedures: Train them on system maintenance and updates.
For instance, a NAITIVE client reported a 34% boost in customer retention and a 41% increase in conversion rates after implementing a Voice AI Agent solution capable of handling 200 outbound calls daily [2].
5.3 Monitor and Update
Once your systems are integrated and your team is trained, regular monitoring becomes essential to keep everything running smoothly. Set up a structured review process with these steps:
- Compare predicted costs to actual results.
- Pinpoint and investigate any major variances.
- Adjust model parameters as needed.
- Ensure data quality remains high.
- Refine forecasting assumptions based on new insights.
Consider using a performance monitoring framework like this:
Metric | Target Range | Review Frequency |
---|---|---|
Forecast Accuracy | ±10% | Weekly |
Data Quality Score | >95% | Daily |
System Uptime | >99.9% | Continuous |
Model Drift | <5% | Monthly |
6. Tips for Better Forecasting
To improve the accuracy of your forecasts after setting up your systems and models, consider these essential tips:
6.1 Use Current Data
Keep your data updated regularly - this includes usage metrics, cost trends, performance stats, and logs. Staying current ensures your forecasts reflect all relevant cost factors and avoid drifting off course.
"The AI Agent NAITIVE designed now manages 77% of our L1-L2 client support." - Sarah Johnson, CXO
6.2 Include All Expenses
Don’t overlook less obvious costs. Be sure to account for areas like data storage, model training, system maintenance, and integrations. These often-overlooked expenses can significantly impact your overall forecast.
"The insights gained from NAITIVE AI advisory sessions has been enlightening." - Sofie, CEO
6.3 Add Market Data
Incorporate external market data alongside your internal metrics to fine-tune your forecasts. Consider these factors:
- Industry benchmarks: AI costs can vary by as much as 45%, so understanding where you stand is critical.
- Economic indicators: Keep an eye on trends in cloud services, hardware costs, and labor rates.
- Technology trends: Stay informed about new AI technologies and their potential cost implications.
"The Voice AI Agent Solution NAITIVE implemented is from the future." - John, CEO
Conclusion: Setup Checklist Summary
Based on the checklist above, your AI cost forecasting system should now be well-structured. These systems rely on three main elements to predict costs effectively and improve ROI:
- Data Foundation: Accurate forecasting depends on strong, complete data. Set up systems to collect all cost-related details, from infrastructure spending to operational overhead.
- Tool Integration: Choose and implement forecasting tools that align with your business needs. These tools should simplify workflows, improve prediction accuracy, and reduce manual workloads.
- Continuous Optimization: Regular updates and improvements are essential to maintaining accuracy. As Sarah Johnson, CXO, highlights:
"The AI Agent NAITIVE designed now manages 77% of our L1-L2 client support."
By following this structured approach, your organization can stay in control of costs while making the most of its AI investments. The focus should remain on adapting to changes and aligning with your core business goals.
Use this checklist as a roadmap to keep your AI cost management efforts on track.