10 Metrics for AI Service Optimization
Explore 10 essential metrics for optimizing AI services, enhancing efficiency, reducing costs, and boosting user satisfaction.
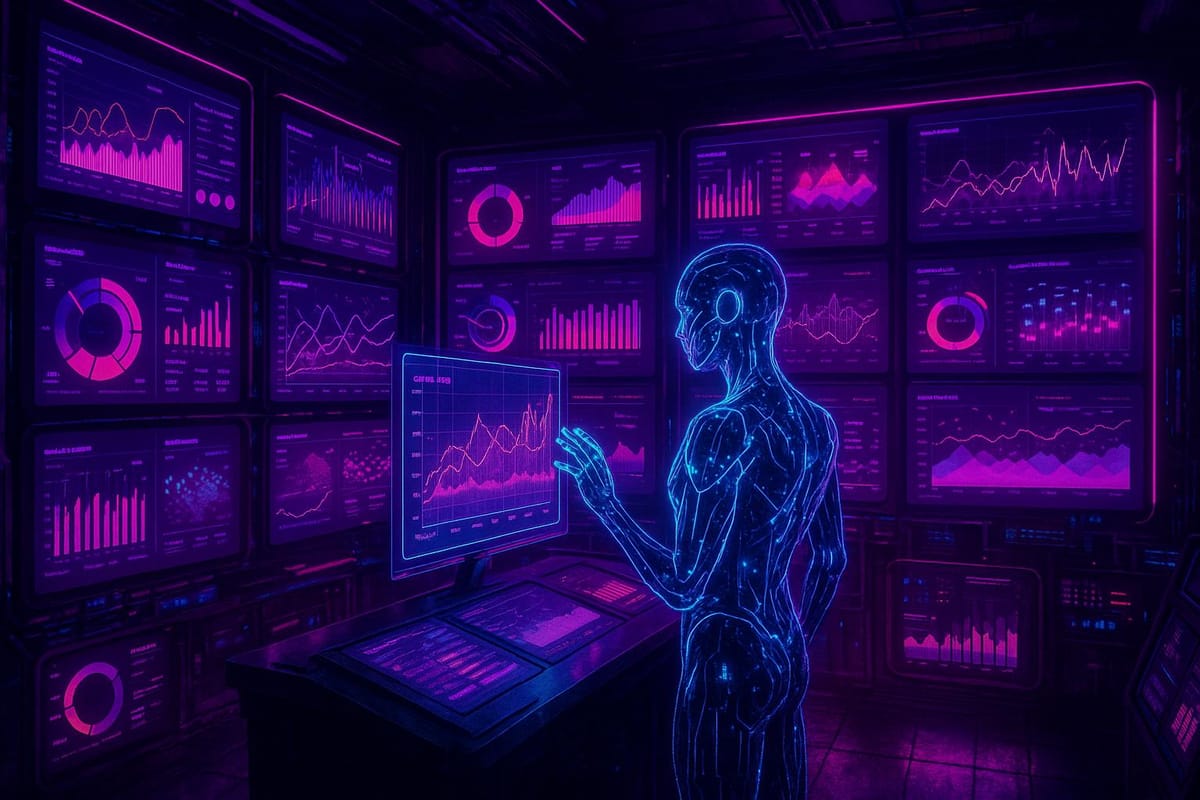
Optimizing AI services is all about measuring the right metrics. Here are the 10 key metrics you need to track to improve performance, reduce costs, and enhance user satisfaction:
- 1. Average Time to Fix Issues (MTTR): Tracks how quickly AI resolves problems. AI systems average 4.2 hours, compared to 7.8 hours for traditional methods.
- 2. One-Contact Problem Resolution Rate: Measures how often issues are resolved in the first interaction. Aim for 70–80% or higher to improve efficiency and customer satisfaction.
- 3. AI Decision Accuracy: Focuses on precision, recall, and F1 scores to ensure reliable AI decisions. Top-performing systems achieve 85–95% accuracy.
- 4. User Satisfaction Scores: Captures how users perceive the AI service through surveys like CSAT and NPS. High scores improve retention and revenue.
- 5. System Processing Speed: Metrics like response time and latency ensure fast and efficient operations. Target speeds vary by industry.
- 6. Service Agreement Success Rate: Tracks adherence to performance targets like uptime, accuracy, and resolution rates.
- 7. Cost Per AI Interaction (CPAI): Evaluates the average cost of handling service requests. Optimizing this cuts expenses while maintaining quality.
- 8. Error Reduction Rate (ERR): Measures how much AI reduces operational errors, improving reliability and trust.
- 9. Self-Service Success Rate (SSR): Tracks how effectively AI resolves issues without human help. High SSR reduces call center volume and costs.
- 10. User Effort Rating (UER): Assesses how easy it is for users to resolve issues with AI. Lower effort equals higher customer loyalty.
Quick Comparison Table:
Metric | What It Measures | Target/Benchmark | Impact |
---|---|---|---|
MTTR | Time to resolve issues | 4.2 hours (AI); 7.8 (manual) | Faster issue resolution |
One-Contact Resolution | First-contact resolution rate | 70–80%+ | Higher efficiency, satisfaction |
AI Decision Accuracy | Precision, recall, F1 score | 85–95% | Reliable decisions |
User Satisfaction Scores | CSAT, NPS | 4.0–4.3 (out of 5) | Retention, revenue growth |
System Processing Speed | Response/latency | Varies by industry | Faster operations |
Service Agreement Success | Uptime, accuracy | 95–99.9% | Reliability, trust |
Cost Per AI Interaction | AI service cost | $0.10–$10 (varies) | Cost savings |
Error Reduction Rate | Error decrease | 30–50% | Improved reliability |
Self-Service Success Rate | Issues resolved by AI | 60–90%+ | Reduced escalations |
User Effort Rating | Ease of issue resolution | 2.0–3.0 (low effort) | Loyalty, satisfaction |
These metrics provide a roadmap to fine-tune your AI systems, enhance performance, and improve customer experiences. Start tracking them today to unlock better results!
AI: Revolutionizing Customer Service & KPIs
1. Average Time to Fix Issues (MTTR)
Mean Time to Repair (MTTR) is all about measuring how quickly systems can identify, diagnose, and fix operational issues. For AI systems, this metric is a big deal - it directly influences system uptime and user experience. The faster issues are resolved, the smoother the service.
AI systems have a clear edge here. On average, they resolve issues in 4.2 hours, compared to 7.8 hours for traditional methods. This speed comes from AI's ability to quickly spot patterns and leverage historical data.
Here’s a breakdown of how different industries benefit:
Industry | Traditional MTTR | AI-Optimized MTTR | Improvement |
---|---|---|---|
Financial Services | 6.3 hours | 2.8 hours | 56% |
Healthcare | 8.7 hours | 5.1 hours | 41% |
Manufacturing | 12.4 hours | 7.1 hours | 43% |
For example, a Fortune 500 financial services company used an AI-powered Resolution Intelligence system to slash its MTTR from 6.3 hours to 2.8 hours. This change saved them around $1.2 million annually and increased customer satisfaction by 23%.
Key Components of MTTR
To effectively reduce MTTR, focus on these three stages:
- Detection Time: How fast the system spots an issue.
- Diagnosis Time: The time it takes to figure out what’s causing the problem.
- Resolution Time: The duration needed to fix and confirm the solution.
Tips for Improving MTTR
Want to bring down your MTTR? Here are some practical steps:
- Use monitoring tools with early warning capabilities.
- Set up automated recovery processes.
- Develop detailed incident response playbooks.
- Build a knowledge base for quick reference.
- Define clear escalation paths to avoid delays.
AI-powered predictive maintenance can be a game changer here. Some organizations have cut MTTR by up to 50% by catching and fixing potential issues before they escalate.
If you're ready to take your AI operations to the next level, NAITIVE AI Consulting Agency (https://naitive.cloud) offers tailored solutions to help you improve metrics like MTTR and streamline your service delivery.
2. One-Contact Problem Resolution Rate
The One-Contact Problem Resolution Rate reflects how effectively an AI system resolves issues during the first interaction. This metric is crucial for boosting service efficiency and keeping customers happy.
Industry Benchmarks
Most organizations aim for first-contact resolution rates of 70–80% or higher, regardless of their industry. These benchmarks serve as a solid starting point for improving performance in practical scenarios.
Real-World Impact
A telecommunications company showcased the value of prioritizing this metric. By implementing advanced AI solutions, they achieved the following:
- First-contact resolution rate jumped from 65% to 82%
- Call center workload dropped by 20%
- Customer satisfaction scores increased by 15%
- Annual cost savings were substantial
These outcomes highlight how focusing on resolution rates can drive meaningful improvements.
Key Factors Influencing Resolution Rates
Data Integration: Success depends on tracking essential data points, such as:
- Interaction timestamps
- Escalation records
- Resolution outcomes
- Contact channels used
- Types of issues reported
AI Model Training: Regular updates and adjustments to AI models are critical to sustaining high resolution rates.
Measuring Success
The formula for calculating this metric is straightforward:
(First-contact resolutions ÷ Total interactions) × 100.
For instance, if an AI system handles 1,000 inquiries in a month and resolves 800 of them on the first attempt, the resolution rate would be 80%.
Tips to Improve Performance
- Analyze Escalation Trends: Pinpoint recurring issues that lead to escalations.
- Keep Knowledge Bases Updated: Ensure the AI system has access to up-to-date solutions.
- Enhance AI Understanding: Improve natural language processing to tackle more complex queries.
- Implement Smart Routing: Assign challenging cases to specialized AI models or human agents.
- Leverage Expert Guidance: Collaborate with AI specialists, like NAITIVE AI Consulting Agency (naitive.cloud), to create tailored strategies for improving first-contact resolution.
Striking a balance between high resolution rates, response accuracy, and overall customer satisfaction is key to delivering exceptional service.
3. AI Decision Accuracy
AI decision accuracy is a critical factor in ensuring your service's reliability. It directly influences the quality of service and operational efficiency by measuring how often an AI system makes the right calls and provides accurate responses during interactions.
Breaking Down the Metrics
To evaluate decision accuracy, focus on these key components:
- Precision: How accurate the AI is with its positive predictions.
- Recall: Its ability to identify all relevant cases.
- F1 Score: A balance between precision and recall.
- Confidence Score: The AI's level of certainty in its decisions.
These metrics are essential for integrating accuracy into your performance evaluation framework.
Real-World Applications
Monitoring decision accuracy in real time helps identify gaps and improve outcomes. For AI-driven customer service, pay attention to:
- Response correctness: How often the AI provides accurate information.
- Solution relevance: Whether the solutions offered effectively resolve customer issues.
- Error patterns: Recurring mistakes that might point to deeper problems.
- Decision confidence: Situations where the AI should escalate to a human agent.
Measuring Accuracy
You can calculate decision accuracy using this formula:
(Correct decisions ÷ Total decisions) × 100.
Additional tools to measure success include:
- Real-time monitoring: Dashboards that track performance continuously.
- User feedback: Customer ratings after AI interactions.
- Outcome tracking: Assessing how effective the solutions are.
Industry Benchmarks
Across industries, top-performing AI systems typically achieve accuracy rates between 85% and 95%, depending on the complexity of the tasks. For high-stakes areas like financial transactions or medical advice, a 98% or higher accuracy rate is often the goal.
Reaching these benchmarks paves the way for ongoing improvements in AI performance.
Boosting Decision Accuracy
Maintaining high accuracy requires consistent effort. Businesses can take these steps:
- Update models regularly: Incorporate new data and scenarios as they arise.
- Train on edge cases: Prepare the AI for rare or unusual situations.
- Use high-quality data: Ensure datasets are clean, current, and unbiased.
- Monitor performance: Set alerts for when accuracy drops below acceptable thresholds.
The Cost of Inaccuracy
Low accuracy doesn't just frustrate users - it increases costs, damages your brand, and lowers satisfaction. On the flip side, high accuracy reduces errors, cuts costs, and improves overall service quality.
Investing in expert AI support can make a significant difference in achieving and maintaining high decision accuracy.
4. User Satisfaction Scores
User satisfaction scores provide a direct window into how well your AI services align with user expectations. While technical and operational metrics are crucial, these scores uniquely capture the user experience and perception of your service.
Measuring Feedback
Gathering user feedback is essential, and there are several effective methods to do so:
- Post-interaction surveys: Collect immediate impressions after a user interaction.
- Customer Satisfaction (CSAT) scores: Use monthly surveys to gauge satisfaction levels.
- Net Promoter Score (NPS): Conduct quarterly assessments to measure loyalty and likelihood of recommendations.
- In-app feedback tools: Capture real-time insights while users engage with your service.
Industry Benchmarks
To put your satisfaction scores in perspective, compare them against industry standards. Here's how different sectors typically perform:
Industry | Average Score (out of 5) | Satisfaction Rate |
---|---|---|
Financial Services | 4.2 | 85% |
Healthcare | 4.0 | 80% |
Retail | 4.3 | 82% |
Telecommunications | 3.9 | 78% |
Travel | 4.1 | 83% |
Impact on Business Metrics
High user satisfaction isn't just a feel-good metric - it directly influences critical business outcomes. Here are some key benefits tied to strong satisfaction scores:
- Improved retention: A 10–15% boost in customer retention rates.
- Revenue growth: An increase of 2–3% in overall revenue.
- Enhanced customer lifetime value: Users may deliver 3–7 times greater lifetime value.
- Cost savings: Support expenses can decrease by 20–40%.
Analysis and Action
To make the most of satisfaction data, it's important to go beyond just collecting scores. Dive deeper by:
- Breaking down feedback by user demographics and types of interactions.
- Analyzing satisfaction trends at different stages of the customer journey.
- Identifying specific AI features that users appreciate or find frustrating.
- Monitoring how satisfaction changes after system updates or new feature rollouts.
Response Protocol
A strong response strategy ensures that user concerns are addressed promptly and effectively:
- Acknowledge feedback: Respond within 24–48 hours.
- Categorize issues: Prioritize based on severity.
- Route concerns: Direct issues to the appropriate team immediately.
- Analyze root causes: Investigate underlying problems thoroughly.
- Follow up: Confirm resolution with the user.
5. System Processing Speed
System processing speed plays a crucial role in determining how efficiently your AI service operates. This is typically measured using metrics like requests per second (RPS), transactions per minute (TPM), or response time. These figures give a clear picture of your system's core performance. Here's a quick breakdown of target speed ranges for different applications:
Application Type | Speed Metric | Target Range |
---|---|---|
Customer Service AI | Response time | Under 1–2 seconds |
Financial AI | Processing latency | 50–200 milliseconds |
Healthcare AI | Processing time | 5–30 seconds |
E-commerce AI | Response time | 200–500 milliseconds |
Manufacturing AI | Items processed | 10–50 items/second |
Hardware and Infrastructure Matter
The speed at which your system processes data depends heavily on factors like hardware setup, network bandwidth, how well the load is distributed, and the optimization of your database.
How to Improve Processing Speed
To hit those performance benchmarks, consider these strategies:
- Intelligent Caching: Store frequently accessed responses to cut down on processing time.
- Progressive Loading: Deliver quick initial results while loading additional details in the background.
- Peak Performance Monitoring: Keep an eye on high-traffic times and use auto-scaling to handle demand surges.
These steps can help you achieve consistent, scalable performance.
Tools to Keep an Eye on Performance
To maintain optimal speeds, leverage tools like:
- Application Performance Monitoring (APM) platforms
- Cloud provider monitoring services
- Real User Monitoring (RUM) systems
- Custom telemetry solutions
Why Processing Speed Matters for Business
System speed doesn’t just affect technical performance - it directly impacts your business outcomes. Here’s how:
Metric | Business Impact |
---|---|
Customer Satisfaction | Faster responses mean happier customers. |
Operational Costs | Optimized speed reduces resource consumption. |
Service Scalability | Better speed allows handling of peak traffic loads. |
Error Rates | Lower latency leads to fewer timeouts and errors. |
6. Service Agreement Success Rate
Service Agreement Success Rate is a key metric that evaluates how well AI systems meet their contractual performance obligations. It measures critical factors such as uptime, response time, accuracy, resolution rates, and adherence to data processing standards.
Key Components to Track
Ensuring success in service agreements relies on tracking specific performance targets and understanding their impact on business outcomes. Below are the core components:
Component | Target Range | Business Impact |
---|---|---|
System Uptime | 99.9%+ | Ensures reliability and service availability |
Response Time | Under 2 seconds | Enhances user experience and satisfaction |
Accuracy Rate | 95-98% | Builds trust through quality decisions |
Resolution Rate | 90-95% | Demonstrates problem-solving efficiency |
Data Processing | 98%+ compliance | Drives operational effectiveness |
Industry-Specific Benchmarks
Performance expectations vary by industry, with leading AI systems achieving the following benchmarks:
AI Application | Success Rate Target | Critical Metrics |
---|---|---|
Customer Service | 95-98% | Response time, query resolution |
Financial Services | 99.9%+ | Transaction accuracy, fraud detection |
Healthcare | 98%+ | Diagnostic accuracy, processing speed |
Manufacturing | 98%+ | Quality control, inspection timing |
Monitoring Best Practices
To maintain compliance with service agreements, consider the following strategies:
-
Real-Time Performance Tracking
Implement automated systems to monitor metrics continuously, enabling immediate detection of issues. -
Proactive Alert Systems
Set up early warning mechanisms to identify and address performance declines before they impact service levels. -
Regular Performance Reviews
Conduct monthly evaluations of AI performance against agreed metrics to uncover trends and areas for improvement.
Impact on Business Outcomes
Achieving high success rates in service agreements directly contributes to better customer retention, streamlined operations, and increased revenue.
"The AI Agent NAITIVE designed now manages 77% of our L1-L2 client support." – Sarah Johnson, CXO
Common Challenges and Solutions
-
Model Drift and Scaling
Combat model drift and scaling challenges with regular retraining, thorough load testing, and dynamic resource allocation. -
Data Quality
Maintain high performance by enforcing strict data validation processes and adhering to quality standards.
7. Cost Per AI Interaction
Cost Per AI Interaction (CPAI) evaluates the average expense of handling a service request through AI. Breaking down the cost components is crucial to understanding and managing CPAI effectively.
Core Cost Components
Here’s a look at the major factors that influence CPAI:
Component | Description | Impact |
---|---|---|
Development | Building and customizing AI models | Upfront |
Infrastructure | Cloud computing and storage costs | Usage-based |
Licensing | Fees for platforms and tools | Recurring |
Maintenance | Updates and ongoing support | Operational |
Human Oversight | Monitoring and managing systems | Overhead |
Industry Benchmarks
Different industries have varying CPAI ranges based on their specific needs and applications:
Industry | Cost Range | Value Comparison |
---|---|---|
Customer Service | $0.10 - $1.00 | Compared to $5-$15 for human agents |
Healthcare | $2.00 - $10.00 | Supports efficient diagnostics |
Financial Services | $0.50 - $3.00 | Enables fraud detection and underwriting |
Retail | $0.01 - $0.20 | Powers high-volume recommendation engines |
Cost Optimization Strategies
Optimizing CPAI is all about finding the right balance between resource use and maintaining service quality. Here are some effective approaches:
- Auto-scale cloud resources to align with real-time demand.
- Perform regular performance audits to identify inefficiencies.
- Optimize token usage to reduce unnecessary processing costs.
- Simplify processing paths for faster, more efficient workflows.
- Boost interaction volume to spread fixed costs across more transactions.
Monitoring Framework
To keep CPAI under control, track these key metrics alongside it:
- System uptime to ensure reliability.
- Response time averages for measuring speed.
- Resolution effectiveness to gauge accuracy.
- Customer satisfaction scores to assess user experience.
- Token efficiency rates to monitor resource usage.
Additional Considerations
When managing CPAI, don’t overlook these factors:
- Security and compliance to meet regulatory standards.
- Data storage and processing for scalability.
- Model retraining cycles to maintain performance.
- Integration maintenance to ensure seamless operations.
8. Error Reduction Rate
The Error Reduction Rate (ERR) takes the idea of improving service quality a step further by focusing on operational mistakes. While metrics like MTTR and decision accuracy help measure efficiency, ERR specifically quantifies how much errors have decreased, giving a clearer picture of how dependable the system has become. Fewer errors mean smoother operations and a stronger foundation of trust in your processes.
Here’s how you calculate ERR:
Error Reduction Rate (%) = [(Errors Before AI - Errors After AI) / Errors Before AI] × 100
Key Error Categories
Identifying and categorizing errors helps pinpoint where AI makes the biggest impact. Below are the main types of errors and their areas of influence:
Error Type | Description | Impact Areas |
---|---|---|
Data Entry | Incorrect information input | Data accuracy, processing time |
Classification | Misidentified items or requests | Service routing, resolution time |
Transaction | Failed or incorrect processes | Customer satisfaction, revenue |
Compliance | Regulatory requirement breaches | Legal risk, penalties |
Response | Inaccurate service delivery | Customer experience, support costs |
Performance Monitoring
To ensure sustained improvement, it’s essential to monitor performance closely. Reviewing system logs can uncover recurring error patterns, while quality assurance processes help track how quickly and accurately the model identifies and addresses errors. These insights can also reveal how error trends affect customer satisfaction and operational costs.
Optimization Strategies
Want to make the most of your AI's error-reduction capabilities? Here are some strategies to consider:
- Set up systems for continuous monitoring to catch issues early.
- Regularly retrain AI models with fresh, updated data.
- Develop a clear error classification framework for easy tracking.
- Keep detailed error logs to analyze trends over time.
- Use automated tools to detect and address errors efficiently.
Looking for tailored solutions? NAITIVE AI Consulting Agency (https://naitive.cloud) can help design strategies that improve AI performance and reduce errors. By focusing on error reduction, you not only improve reliability but also enhance overall user satisfaction - key to long-term success.
9. Self-Service Success Rate
Measuring self-service success is a key step in understanding how well your AI system performs. The Self-Service Success Rate (SSR) evaluates how effectively your AI resolves issues without needing human assistance. This metric plays a big role in improving efficiency and cutting costs.
How to Calculate Self-Service Success Rate
Here’s the formula:
SSR (%) = (Issues Resolved by AI / Total Self-Service Interactions) × 100
For example, if your AI handles 1,000 customer queries and successfully resolves 800 without needing to escalate to a human, your SSR would be 80%.
Performance Benchmarks
Different industries and use cases set varying targets for SSR. Here’s a quick breakdown:
Complexity Level | Target SSR | Use Cases |
---|---|---|
Basic | 90%+ | FAQ responses, account status checks |
Intermediate | 70-85% | Billing questions, product support |
Complex | 60-70% | Technical troubleshooting, custom requests |
Real-World Impact
Improving SSR can lead to tangible benefits. For instance, raising self-service success from 60% to 85% has been shown to deliver:
- A 30% drop in call center volume
- Quicker resolution times
- Higher customer satisfaction scores
These gains open the door to further fine-tuning and efficiencies.
Strategies to Improve SSR
Boosting your AI’s self-service capabilities requires thoughtful strategies, such as:
- Continuous Learning: Leverage real interaction data to improve your AI’s accuracy over time.
- Intent Mapping: Regularly refine your AI’s ability to understand and respond to user requests.
- Feedback Integration: Use customer feedback to identify and address weak spots.
- Knowledge Base Updates: Keep your knowledge base current to ensure accurate responses.
Key Metrics to Track
To get a full picture of your AI’s performance, monitor these alongside SSR:
- Accuracy in resolving issues
- User satisfaction levels
- Escalation trends
- Response times
For example, recent projects by NAITIVE AI Consulting Agency highlight the potential of AI-powered self-service. One client saw their AI agent independently handle 77% of L1-L2 support queries, while another reported a 34% boost in customer retention after implementing self-service solutions.
It’s worth noting that effective self-service isn’t just about reducing escalations. The ultimate goal is to provide solutions that not only solve problems but also leave customers feeling satisfied - all while keeping costs in check.
10. User Effort Rating
User Effort Rating (UER) evaluates how much effort customers need to put in when resolving issues using AI. It's a critical metric because it directly influences customer satisfaction and loyalty.
Measuring User Effort
Here’s how UER scores break down:
Score Range | Effort Level | Indicators |
---|---|---|
1.0-2.0 | Minimal | Resolved in one interaction, no hassle |
2.1-3.0 | Low | Quick resolution with minor clarification |
3.1-4.0 | Moderate | Involves multiple steps or some repetition |
4.1+ | High | Requires clarifications or switching channels |
Industry Benchmarks
UER expectations vary by industry, reflecting the complexity of customer interactions:
- Retail/E-commerce: 2.0–2.5
- Financial Services: 2.3–2.8
- Telecommunications: 2.5–3.0
- Healthcare: 2.8–3.2
These benchmarks help businesses gauge their performance and set realistic goals for improvement.
Impact on Business Metrics
Research has shown that reducing effort by just one point can boost customer loyalty by 25–30% while simultaneously cutting churn rates by 10–15%.
Key Factors Behind UER
Several components shape UER and influence its outcomes:
- Cognitive Load: The mental effort required to understand AI responses.
- Resolution Time and Conversation Turns: How long and how many steps it takes to resolve an issue.
- Channel Switching: Whether users need to move between communication methods.
- Emotional Effort: The stress or frustration customers experience during the interaction.
Strategies to Improve UER
NAITIVE AI Consulting Agency has implemented strategies to make AI interactions smoother and more effective, leading to noticeable improvements:
- Advanced Intent Recognition: Leveraging cutting-edge natural language processing to reduce repetitive questions.
- Contextual Memory: Retaining conversation context to avoid asking the same questions repeatedly.
- Proactive Resolution: Addressing potential follow-up questions before they arise.
Businesses that have adopted these practices have seen a 25–40% improvement in their UER scores within 6–12 months.
Ultimately, UER isn’t just about cutting down the number of steps; it’s about creating interactions that feel effortless. Achieving this balance strengthens both operational performance and customer loyalty.
Performance Metrics Comparison
Understanding the interplay between key AI service metrics is essential for making smart optimization decisions. Let’s break down the trade-offs between speed, cost, accuracy, and resolution time to see how they influence performance.
Speed vs. Cost Trade-offs
Delivering faster responses often demands more advanced resources, upgraded infrastructure, and premium AI models. While real-time processing ensures immediate replies - perfect for time-sensitive scenarios - it comes at a higher cost. On the other hand, batch processing, though slower, offers a more budget-friendly option by processing data in bulk and tolerating delays.
Accuracy vs. Resolution Time
Achieving higher accuracy can sometimes mean longer resolution times. For instance, a telecommunications company reduced escalation rates by 30% but saw a 15% increase in handling time. Interestingly, this trade-off worked in their favor - customers appreciated the thorough problem-solving, leading to better satisfaction rates despite the added wait.
Balancing Multiple Metrics
Finding the right balance between speed, cost, accuracy, and resolution time is key to achieving optimal performance. For example, if cutting costs negatively impacts customer satisfaction, it might be worth investing in enhanced infrastructure or more advanced AI models. By understanding these trade-offs, businesses can craft solutions that align with their specific goals. NAITIVE AI Consulting Agency specializes in helping companies navigate these decisions to create tailored AI strategies.
Industry-Specific Considerations
Each industry has its own priorities when it comes to balancing these metrics. Here’s a quick look at how different sectors weigh their options:
Industry | Primary Focus | Secondary Focus | Rationale |
---|---|---|---|
Healthcare | Accuracy | Resolution Time | Ensures patient safety and meets strict regulations. |
Retail | Speed | Cost | Handles high volumes with the need for quick responses. |
Finance | Accuracy | Resolution Time | Critical for compliance and managing risks. |
Performance Optimization Framework
These insights can be combined into a framework to guide decision-making:
- Speed-Cost Ratio: Improving speed by 10% can drive up costs by 15–20%.
- Accuracy-Resolution Balance: Higher accuracy might extend initial resolution times but can reduce repeat interactions, saving time overall.
- Cost-Effectiveness Threshold: Beyond a certain point, additional investments in speed or accuracy may not deliver proportional benefits.
Measuring Success
To ensure success, track real-time KPIs like cost per interaction and customer satisfaction scores. These metrics provide actionable insights, helping businesses fine-tune their AI investments and strategies based on their unique needs and limitations. With this approach, companies can make data-driven decisions to optimize their AI systems effectively.
Conclusion
Keeping a close eye on these ten AI service metrics can help businesses fine-tune their operations, enhance service quality, and manage costs more effectively. For example, the telecommunications industry has seen a 30% boost in containment rates and a 20% drop in average handle time by focusing on balancing these metrics. The results? Tangible, compounded operational improvements.
To make the most of AI service optimization, three key factors come into play:
- Comprehensive Monitoring: It's essential to track both technical performance and user experience metrics. This ensures that improvements are balanced and meaningful across the board.
- Strategic Trade-offs: Understanding how metrics interact is critical. For instance, improving AI accuracy may initially lead to longer resolution times, but it can also reduce repeat issues and improve customer satisfaction in the long run.
- Continuous Refinement: Regularly reviewing and adjusting metrics ensures that AI services stay aligned with changing business goals and customer needs.
As discussed earlier, focusing on technical, operational, and user-centric metrics creates a full-circle approach to optimization. A great example of this is NAITIVE AI Consulting Agency's Voice AI Agent Solution, which achieved notable gains in handling support queries and retaining customers by leveraging metric-driven strategies.
FAQs
How can businesses find the right balance between speed, cost, and accuracy in AI systems to improve performance?
Balancing speed, cost, and accuracy in AI systems starts with a clear understanding of your business priorities. Pinpoint which factor - be it speed, cost, or accuracy - matters most for your operations. For instance, healthcare organizations often emphasize accuracy, while e-commerce platforms may lean toward speed to enhance customer service.
Here are some key considerations to help fine-tune performance:
- Data Quality: Better data leads to more accurate results but might require extra processing time and resources.
- Scalability: Opt for solutions that handle growing workloads without driving up costs significantly.
- Testing and Monitoring: Regularly assess system performance to ensure it aligns with your business needs and expectations.
By aligning AI system capabilities with your objectives, you can strike a balance that boosts efficiency and brings measurable benefits to your organization.
How can companies enhance AI decision accuracy, and why is it essential for reliable AI-driven services?
Improving AI decision accuracy is essential for ensuring reliable outcomes, which play a big role in both customer satisfaction and operational success. Here’s how companies can work toward better accuracy:
- Refine training data: Using datasets that are high-quality, diverse, and regularly updated helps minimize biases and boosts the model’s precision.
- Continuous monitoring: Keep track of AI performance with real-world data and make adjustments to the models when necessary.
- Use explainable AI (XAI): Transparent AI decision-making makes it easier to spot and fix errors.
When AI systems make accurate decisions, they not only build trust with users but also cut down on expensive mistakes and elevate the quality of services provided. This makes accuracy a key foundation for any AI-powered service.
How does improving the self-service success rate enhance customer satisfaction and operational efficiency?
Improving the self-service success rate can make a big difference for both customers and businesses. When people can solve their problems quickly and on their own - using tools like AI chatbots or automated phone systems - they enjoy the convenience of not waiting around for help. This not only saves time but also leaves a better impression, which can lead to stronger customer loyalty.
For businesses, better self-service success means less pressure on support teams. With fewer inquiries needing human intervention, companies can use their resources more efficiently, cut costs, and focus on more complex or high-priority issues that require a personal touch. In short, fine-tuning self-service systems creates a win-win situation: happier customers and a more streamlined, cost-effective operation.